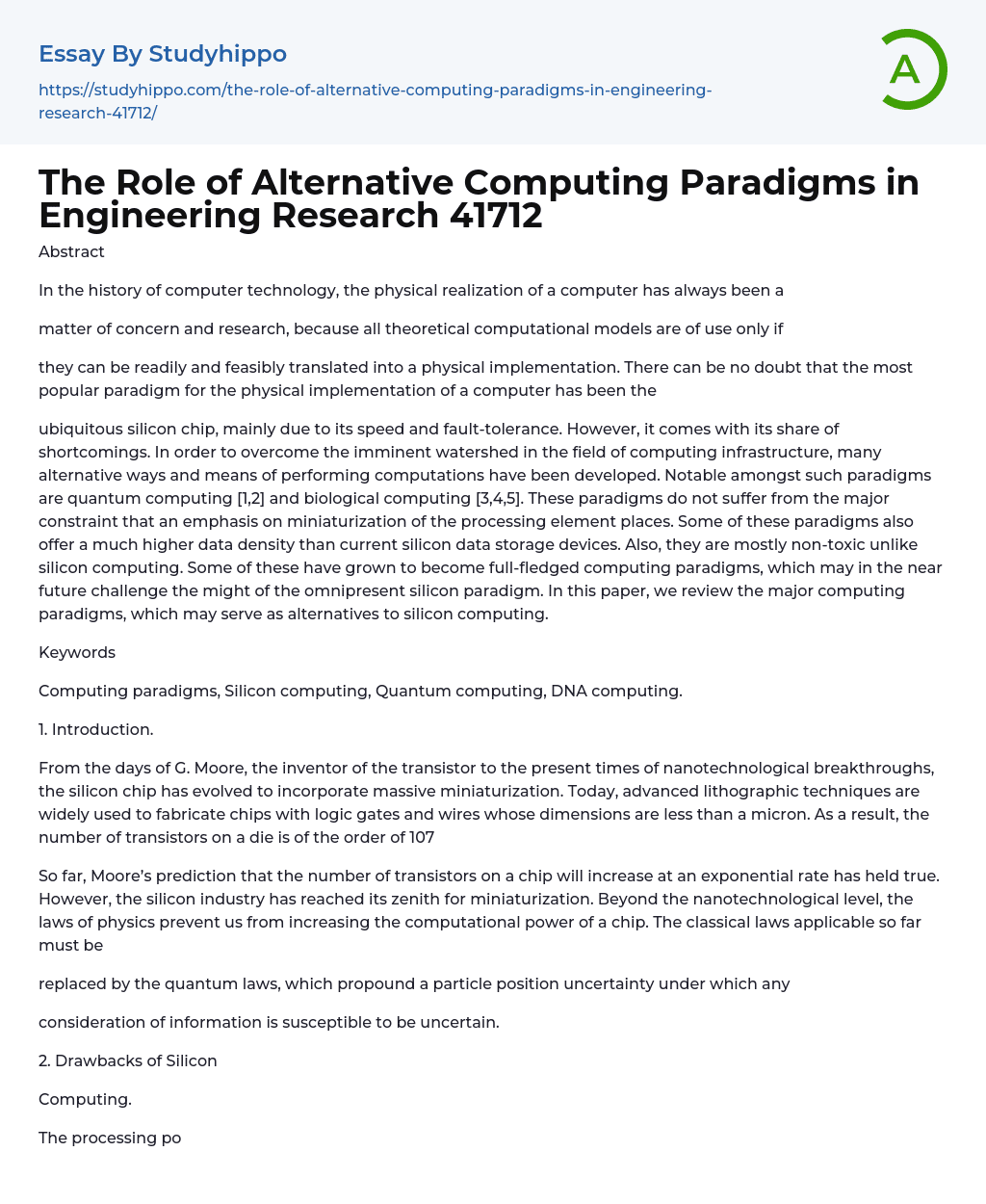
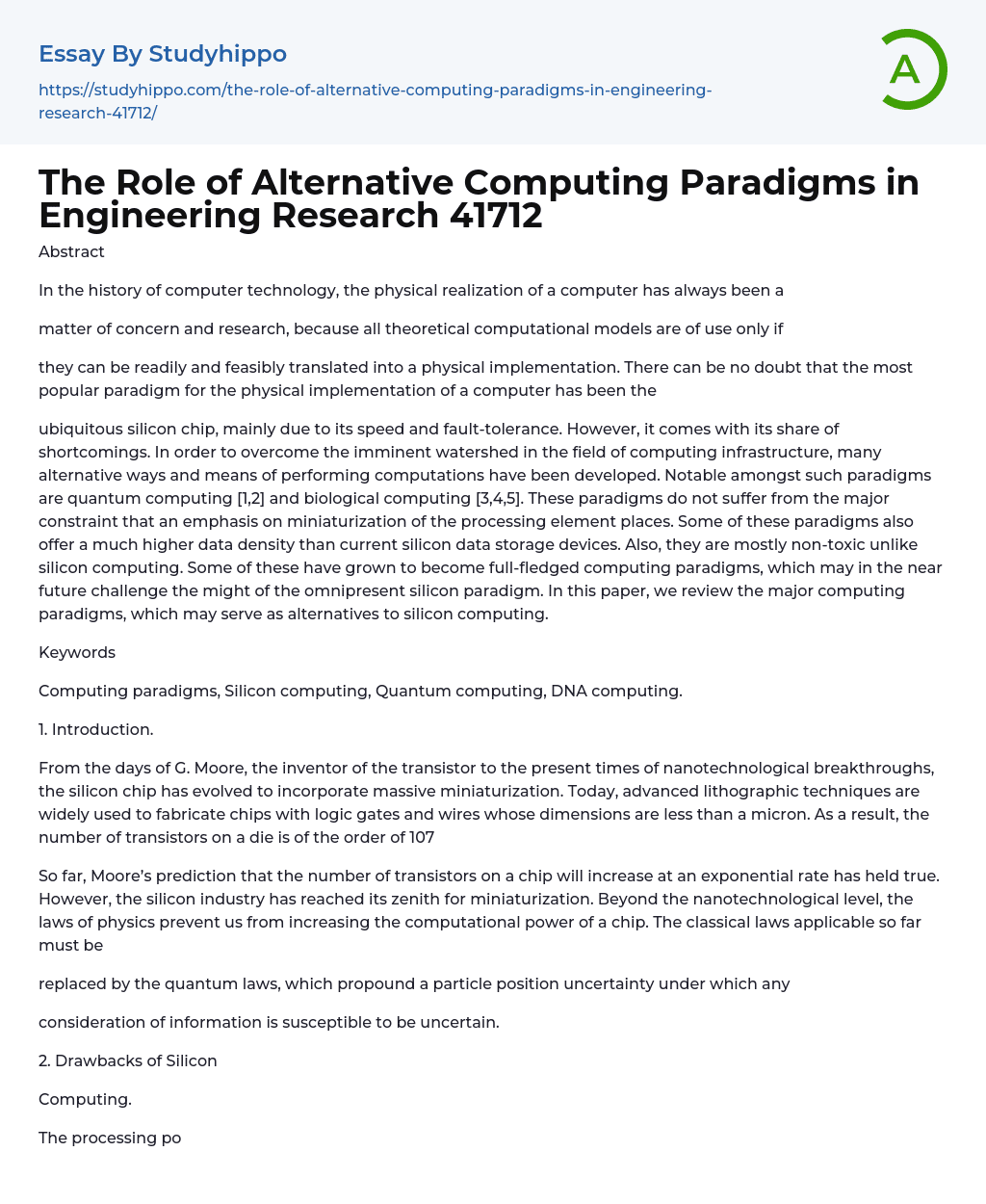
The Role of Alternative Computing Paradigms in Engineering Research 41712 Essay Example
The following is an abstract:
Throughout the history of computer technology, the physical manifestation of a computer has consistently been a
The topic being investigated and studied is the efficacy of various theoretical computational models.
Undoubtedly, the most frequently employed method of physically constructing a computer has been a paradigm that can be easily and realistically transformed into an actual implementation.
Various methods have been developed as alternatives to silicon chips, such as quantum computing [1,2] and biological computing [3,4,5]. These paradigms offer benefits like faster processing speed, fault tolerance, larger data storage capacity, and the use of safe materials. In the future, they may pose a challenge to the current dominance of silicon computing. This article investigates these noteworthy computing paradigms as potential substitutes for traditional silicon-based computation.
Keywords
Computing paradigms such as Silicon computing, Quantum computing, and DNA computing.
...
1. The text begins with an introduction.
Since G. Moore's era, the creator of the transistor, there have been notable advancements in extreme miniaturization of the silicon chip up until today's age of nanotechnological progress. At present, advanced lithographic methods are extensively employed to manufacture chips with logic gates and wires smaller than a micron. Consequently, approximately 107 transistors can be found on a single die.
Moore's prediction about the exponential increase of transistors on a chip has been validated thus far. However, the silicon industry has reached its maximum potential for miniaturization. When we delve into nanotechnology, the laws of physics impede further progress in enhancing computational power for chips. Consequently, conventional laws become irrelevant.
Replacing the classical laws, the quantum laws propose a state of uncertainty regarding the position of particles.
Considering information can be subject to uncertainty.
Silicon has certain disadvantages.
The field
of Computing.
The processing power of silicon-based computing has significant growth potential.
Computers are usually subject to limitations in their capabilities and processing power due to the laws of physics.
Speed measurement in circuits involves determining the rate at which information can be transmitted from point A to point B.
The traditional computing design paradigm accelerates the processing of information once it reaches B by minimizing the distance that electrical signals have to travel. In other words, it aims to reduce the distance between A and B.
B. In order to accommodate additional processing elements or transistors, the central unit has been consistently packed with more and more.
Every transistor within the processing chip of the computer operates as a tiny switch that can be either on or off.
Currently, the density of transistors has reached an astonishing level, with a typical Pentium IV chip being able to hold 55 million transistors in a space equivalent to a dime. This continuous drive towards miniaturization has resulted in significant advancements in computing technology over a relatively short period of time. To provide some perspective, let's compare a desktop computer with a Pentium chip to the ENIAC computer from the 1940's. The ENIAC, which used 17,000 vacuum tubes (the precursor to transistors), weighed 30 tons and occupied an entire room; however, its processing power was less than one hundred thousandth of that.
The Pentium has undergone constant evolution and advancement through innovative techniques to minimize the size of transistors, enabling a greater number of them to fit on a chip. This ongoing progress will ultimately lead to a substantial reduction in size as transistors continue to shrink even further.
Decreasing the size of computers can be
achieved by constructing them with individual atoms or small groups of atoms. However, at this scale, the quantum effects of physics will hinder the efficient transmission of signals. According to the Heisenberg Uncertainty Principle [6], particles (such as electrons that convey information signals in computers) can exhibit peculiar behavior and exist in locations other than their anticipated positions. The exact whereabouts of these particles cannot be fully determined by researchers at any given moment. Consequently, electrons, which should ideally be swiftly traversing atomic-scale circuit pathways in future silicon computers, may instead be found elsewhere along with the information they were carrying.
It is crucial to find viable alternatives to silicon-based technology due to the various problems associated with it. One challenge is the use of hazardous substances such as arsenic in computer processing chips, which creates difficulties in both manufacturing and disposal processes. Additionally, silicon-based computers consume a significant amount of energy.
The inefficient use of energy results in a substantial amount of wastage, both in the form of generated heat and consumed energy.
Now, let us consider various options for the current computing given these restrictions.
paradigm.
Quantum Computing: 3.
In 1982, Feynman made a significant breakthrough in Quantum Computing by demonstrating the possibility of simulating quantum-mechanical objects using other quantum systems [1]. Later, Deutsch from the University of Oxford further advanced this development by introducing a prototype for a Quantum Computer [7].
In 1994, computer scientists became fascinated with the Quantum Computer after Shor created the first quantum algorithm for factorization [8].
Quantum computers outperform all other computers in terms of capabilities.
Quantum computing originated from the concept of utilizing an atom as a bit.
The concept of coherent superposition is about how
an atom can simultaneously be in its excited state and ground state. This is what forms a quantum bit (qubit) [9].
The wave function represents the object's state through mathematical equations.
The complex exponential of the quantum mechanical system contains all possible phases.
If ?1 and ?2 represent the wave functions of any two, the state can be expressed.
According to quantum mechanics, the superposition of two independent states of a system can be represented by the wave function c1?1 + c2?2.
Not all coherent superpositions of two quantum states of a system can maintain stability.
Preserving coherence between the two states used for superposition is crucial in quantum computing, as it ensures stability and enables simultaneous operations on all numbers in a register that is coherent-superpositioned.
In one computational step, a quantum computer can perform an operation on 2L different elements.
The utilization of L qubits, with coherent superpositions, when inputting numbers is equivalent to the computational steps performed by a classical computer. This leads to a noteworthy enhancement in computational resource utilization.
The true potential of quantum computation resides in novel quantum algorithms that enable the utilization
Quantum superposition refers to a state that can hold an exponential amount of distinct terms.
Despite unresolved technical challenges, quantum computing has remained elusive. Nevertheless, methods such as Nuclear Magnetic Resonance have been used to showcase the concepts and principles of quantum computing.
Resonance, Ion Trap, Quantum Dot [10, 11, 12]
Optical Methods and other techniques.
The practical applications of quantum computing cryptography [13] include rapid searching, factorizing large numbers [14], and simulating quantum-mechanical systems with efficiency.
Quantum computing has made several positive advancements since its beginning, including the development of quantum computers with two or three qubits.
At present,
quantum computing can carry out fundamental arithmetic operations and arrange data.
Quantum hardware is still in its early stages and continues to be a growing field of research.
Future progress in quantum computing is expected based on recent advancements.
In the future, larger devices will be available for testing Shor's and other quantum algorithms. This advancement will cause quantum computers to become the leading computational devices. Although the beginnings of quantum computation can be traced back to specialized fields of theoretical physics, its undeniable impact on society is evident in our daily lives. Consequently, there has been a rapid acceleration in worldwide research efforts focused on both the theoretical and experimental facets of quantum computation.
Biological Computing is a scientific discipline that concentrates on utilizing biological systems and processes to carry out computational tasks.
Biological computing utilizes living organisms or their components to execute computing tasks, including storage. Unlike quantum or optical computing, biological computing takes a different approach to overcome the limitations of silicon-based computers. Instead of aiming for faster individual computing operations, biological computing focuses on employing massive parallelism. This involves assigning small portions of a computing task to numerous processing elements. While each element alone may not complete its task rapidly, the large number of such elements working in parallel allows for expedited processing operations.
Silicon-based computers utilize massively parallel processing, yet they cannot reach the same level of parallel processing that biological computers can achieve. The biological functionality of biological computing also makes it ideally suited for overseeing processes that demand an interface between other biological processes and human technology.
4.1 DNA Computers.
DNA computers use DNA strands for computational tasks.
Operations entail the utilization of DNA, which is the
coding substance responsible for storing the instructions for living organisms. DNA comprises four elements.
The DNA amino acids consist of Adenine, Cytosine, Guanine, and Thymine. In DNA, Adenine only pairs with Thymine, while Cytosine only pairs with Guanine. This pairing pattern is consistent and predictable.
Enables the organization of DNA strands into the well-known complete DNA double helix structure;
DNA is well suited to computation due to this specific characteristic.
DNA computers operate by manipulating and disrupting the chemical bonds between molecules to process information.
DNA computing utilizes DNA strands to symbolize problem components.
The structure of DNA resembles the binary code structure in computer language, representing elements of a problem. Numerous unique DNA strands can represent all potential solutions to the problem. These potential solution strands react with problem strands and bind together based on DNA's binding rules. The resulting DNA molecules contain the solutions. Multiple processing steps are undertaken to extract the correct solution from the various possibilities. Finally, the electronic component of the computer analyzes the results.
In silicon-based computers, trillions of reactions can occur simultaneously, mimicking massively parallel processing. This allows for a vast number of potential problem solutions or searches through information pools.
DNA computers can perform answers simultaneously due to their unique mode of functioning.
DNA computers can be used to solve diverse problems, similar to traditional silicon-based computers. This versatility allows them to be classified as true computers according to the Turing machine concept proposed by Turing in 1936.
DNA computing has already demonstrated its value by successfully solving complex logic problems.
[19]. In 1994, Adleman [20] achieved a significant breakthrough by successfully creating and utilizing a
Scientists have developed a DNA computer that can solve the
famous 'traveling salesman' problem.
Using DNA strands to find the most efficient route for a salesman to visit seven cities, passing through each city only once, was a significant showcase of algorithm potential. While a human could quickly complete this task with pen and paper, the utilization of DNA demonstrated its capabilities.
That was only the beginning.
In March 2002, a DNA computer was developed by a team led by L. Adleman, as announced by NASA.
Adleman's work solved a problem that demanded the evaluation of one million alternatives against 24 separate criteria. The objective of his work, and similar efforts, is to enhance the capacity of computers to tackle more complex problems [19], while simultaneously devising methods to minimize errors in the process.
Not all DNA computing follows the format established by Adleman's work. There is a variant of DNA computing.
Computing is being reimagined to mimic the structure of traditional computers.
In 1997, researchers at the University of Rochester created DNA logic gates [11]. Rather than using electrical signals, these gates operate by utilizing DNA inputs and outputs. Their purpose is to identify genetic fragments and merge them to create a single output [21].
In November 2001, a group of Israeli scientists created a range of minuscule DNA computers, totaling trillions in number.
These computers, made of DNA, can fit in a test tube and use DNA software to compute with high reliability [22].
Genetic Algorithms are described in section 4.2.
Any software that utilizes variation and selection to generate an outcome is considered a genetic algorithm.
The output of an evolutionarily tuned program can be a program, a value, or a picture. In order to determine which variants to discard and
which to use, a genetic algorithm requires a process for generating new versions and feedback on their fitness. The problem in a genetic algorithm is encoded as a series of manipulated bit strings.
Artificial Life (Alife) [25] is the field focused on studying computer-generated virtual organisms, often connected to the genetic algorithm.
Virtual organisms typically reside on a virtual grid and can reproduce and consume virtual nutrients. These simulations are sometimes employed to study actual organisms or observe the development of behaviors similar to those found in the real world. By utilizing genetic algorithms to construct our own artificial evolutionary settings, we gain insight into various aspects.
Previously, there were various types of life that only existed in the realm of imagination.
One Alife simulation involves 'organisms' that are simply illuminated pixels that impact the surrounding pixels.
Based on whether adjacent pixels are lit up or not[26], these organisms can create complex high-level effects that were unpredictable just by looking at the low-level components.
Genetic algorithms have the potential to generate innovative solutions to problems that human operators may not have considered due to their ability to explore extensive search spaces. However, this benefit comes with the drawback of computationally intensive processes for problems with large search spaces and a massive number of potential solutions. By combining the strengths of human thinking and the randomized search-and-test approach of genetic algorithms, novel solutions can be developed across various disciplines such as biology, engineering, mathematics, and more.
4.3 Genetic Programs and Genetic
Robots.
Scientists are creating genetic 'computer programs' that could be inserted into and
Living cells can replicate genetic material to control their processes. Researchers have already created engineered sequences of genetic material that
can make a living cell produce one of two genes [27]. This is similar to computer programs. These sequences can act as "switches" to control the chemicals synthesized by living organisms [28]. Scientists are currently working on developing genetic "robots" that include data processing elements, memory storage elements, and communication elements. These robots could reside in cells and enable microscopic interaction with living processes, which is not achievable with silicon-based computing technology.
Such a technique has the potential to give humans an unparalleled level of control over various processes. It could enable individuals to self-manage a range of currently untreatable genetic disorders, while also maintaining a group of miniature "physicians" ready to address any future issues.
4.4 DNA Data Storage.
The experts also highlight the potential as another strong point in favor of biological computing.
The storage capacity of DNA within bacteria is 29.
Successful experiments have allowed for the retrieval of non-native information from a bacterium, ranging from 57 to 99 base pairs.
When considering that a millilitre of liquid can hold 109 bacteria, and assuming an average of 80 base pairs of information per bacterium, the data volume would be 19 Gigabytes using 4 base pairs per byte. In comparison, the current record for magnetic storage is 10 Gigabytes per square inch. However, if we take into account that the hard-disk platter is 1cm thick, the storage density would be 1.5 Gigabytes per millilitre. Therefore, DNA storage within bacteria surpasses traditional storage mediums.
As the volume of data being stored increases, the corresponding amount of overhead required to keep the data organized also increases.
It takes 109 bacteria, 30 bits, or 15 base pairs to specifically identify each bacterium.
The growth rate of the required sequence number space is O(log)2n, which is a considerable portion of the current usable capacity.
Conclusions:
Research in alternative computing paradigms, both experimental and theoretical, is progressing rapidly.
World-wide, there are new proposals for realizing quantum and biological computers.
[30,31], there are constantly new types of alternative computation being discovered and analyzed, which have various advantages over classical computation. We believe that some of these alternative computation methods will lead to technological advancements.
6. References
[1] Feynman R. P., “Miniaturization,” Reinhold Publishing Corporation, New York, pp. 282-296, 1961.
[2] Simon Bone, "An Introduction to Quantum Computers," 1997.
[3] The article titled "An Overview of The Evolutionary Trends in Molecular Computing using DNA" by A. Maurya, A. Nair, and S. Sanyal has been accepted for publication in the International Journal of Computing, Information Technology, And Engineering (IJCITAE) in December 2007.
The citation for "Evolutionary Computing" edited by Corne D. and Shapiro J. L. is as follows: [4] Corne D., and Shapiro J. L., editors, "Evolutionary Computing," volume 1305, Lecture Notes in Computer Science, Springer-Verlag, 1997.
[5] Paun, G., Rozenberg, G., Salomaa, A. "DNA Computing: New Computing Paradigms." Texts in Theoretical Computer Science, EATCSSeries, Springer-Verlag, 2006.
[6] Heisenberg, "Uber den anschaulichen Inhalt der quantentheoretischen Kinematik und seine weiteren Entwicklungen"
Mechanik, Zeitschrift fur Physik, 1927, pp. 172-198. English translation: J. A. Wheeler and
H. Zurek's book "Quantum Theory and Measurement" was published by Princeton University Press in 1983 and covers pages 62-84.
In his book "The Fabric of Reality: The Science of Parallel Universes - and Its", David Deutsch discusses the idea of parallel universes.
The text "Implications," is from the book The Penguin Press, 1997 (6).
[8] David Harel, "Algorithmics: The Spirit of Computing," Addison-Wesley, 2nd
edition, 1992.
[9] In this paper, the topic of Josephson Persistent-Current is discussed by J. E. Mooij, T. P. Orlando, L. Levitov, L. Tian, and C. H. Van.
The information is from "Qubit-Science," published in 1999.
[10] The publication date of the book "Principles of Nuclear Magnetic Resonance in One and Two Dimensions" is 1990.
The article "A Quantum Revolution for Computing" by R. R. Ernst, G. Bodenhausen, A. Wokaun, and J. Brown was published in New Scientist in 1994 [11].
An article on quantum computation was published in Science Volume 270 [12] by David P. DiVincenzo in 1995.
[13] Ekert, "Quantum Cryptoanalysis-Introduction," http://eve.physics.ox.ac.uk/QCresearch/cryptoanalysis/qc.html
[14] Ivars Peterson wrote an article titled "Quantum - Quick Queries" in Science News Volume 150, 1996.
[15] Wong, Wong, and Foote published the article "Organic Data Memory – Using the DNA Approach" in the Communications of the ACM.
2003.
[16] R. J. Lipton, "Speeding up computations via Molecular Biology," Princeton University
Draft from 1994.
[17] Powledge T., "The Polymerase Chain Reaction," http://www.faseb.org/opa/bloodsupply/pcr.html
[18] Turing A. M., "On Computable Numbers, with an Application to the Entscheidungs problem,"
1936.
[19] J. C. Cox, D. S. Cohen, A. D. Ellington, "Trends in Biotechnology - The complexities of
DNA Computation was published in 1999.
[20] Adleman, L.M., "Molecular Computation of Solutions to Combinatorial Problems," Science,
266: 1021- 1024, November 11, 1994.
[21] Weiss R. & Basu S., "The Device Physics of Cellular Logic Gates," First Workshop on Non-
Silicon Computing, based in Cambridge, Massachusetts, was established in February 2002, and operated until 1961.
[22] Elowitz M. and Leibler S., "A Synthetic Oscillatory Network of Transcriptional Regulators," Nature, 403:335-338, January 2000.
Below is aand unified version of the given text, retaining the and their contents:
[23] M. Mitchell, “An Introduction to Genetic Algorithms,” 1996.
[24]
Belew R. K. and Vose M. D., editors, “Foundations of Genetic Algorithms – 4,” pages 117 – 139, Morgan Kaufmann, 1997.
[25] Mitchell, M., & Forrest, S. (1994). "Genetic Algorithms and Artificial Life."
[26] Levy S., “Artificial Life,” ISBN: 0224035991, Cape, 1992.
[27] Schena M., Shalon D., Davis R. W., Brown P. O., "Quantitative Monitoring of Gene"
The text is a citation for a scientific paper titled "Expression Patterns with a Complementary DNA Microarray" which was published in the journal Science in October 1995, volume 270, issue 5235, pages 467-470.
[28] Gardner T., Cantor R., and Collins J., "Construction of a Genetic Toggle Switch in Escherichia Coli," Nature, 403:339 - 342, January 2000.
[29] "Data Stored in Multiplying Bacteria," NewScientist, 2003, http://www.newscientist.com/news/news.jsp?id=ns99993243
[30] J. Khodor, D. K. Gifford, "The Efficiency of Sequence-specific Separation of DNA Mixtures"
for Biological Computing," 3rd Annual DIMACS Workshop on DNA Based Computers.
[31] "Quantum Code-breaking," The Economist, 30
April 1994.
- Computer File essays
- Desktop Computer essays
- Servers essays
- Animals essays
- Charles Darwin essays
- Agriculture essays
- Archaeology essays
- Moon essays
- Space Exploration essays
- Sun essays
- Universe essays
- Birds essays
- Horse essays
- Bear essays
- Butterfly essays
- Cat essays
- Dolphin essays
- Monkey essays
- Tiger essays
- Whale essays
- Lion essays
- Elephant essays
- Mythology essays
- Time Travel essays
- Discovery essays
- Thomas Edison essays
- Linguistics essays
- Journal essays
- Chemistry essays
- Biology essays
- Physics essays
- Seismology essays
- Reaction Rate essays
- Roman Numerals essays
- Scientific Method essays
- Mineralogy essays
- Plate Tectonics essays
- Logic essays
- Genetics essays
- Albert einstein essays
- Stars essays
- Venus essays
- Mars essays
- Evolution essays
- Human Evolution essays
- Noam Chomsky essays
- Methodology essays
- Eli Whitney essays
- Fish essays
- Dinosaur essays