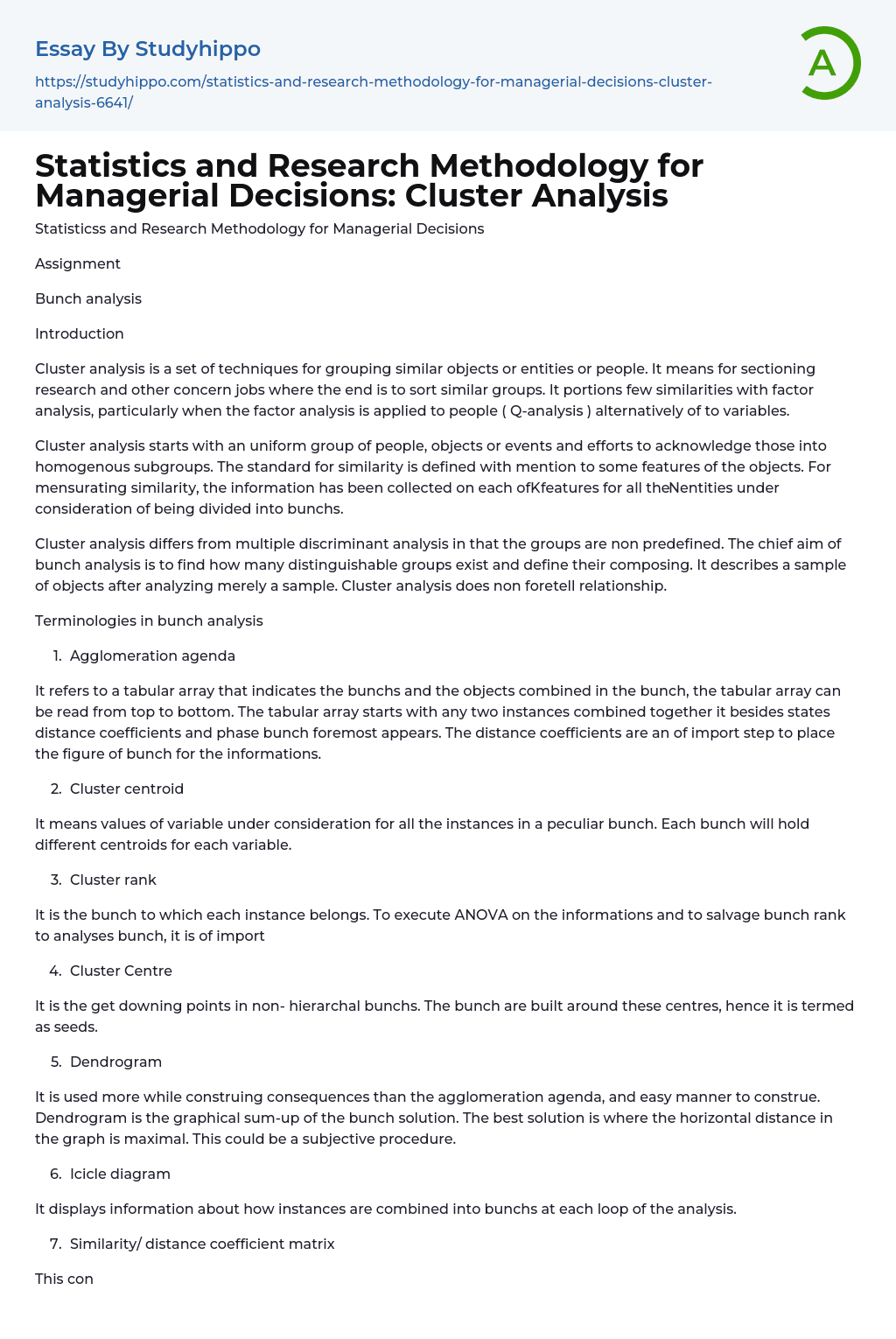
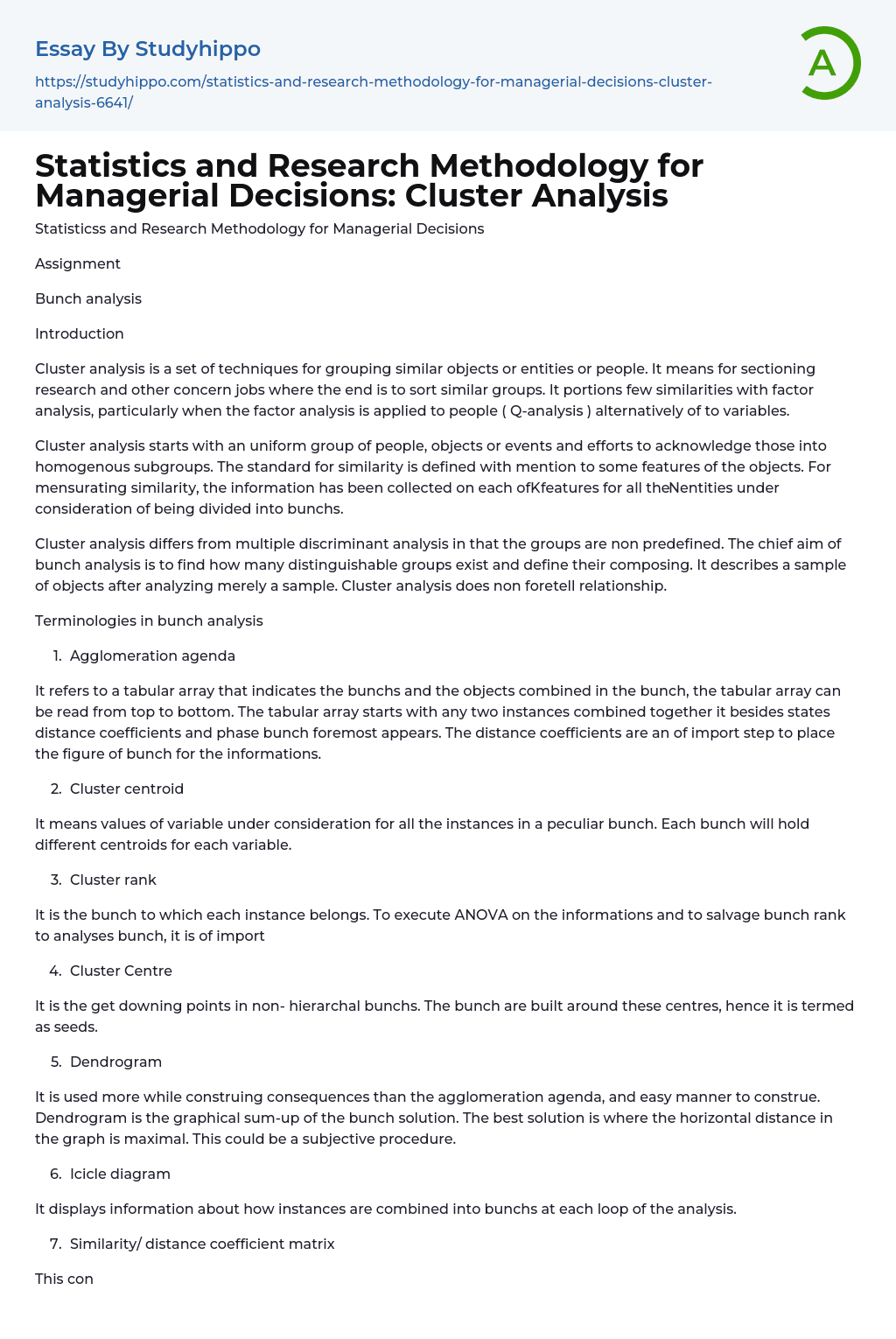
Statistics and Research Methodology for Managerial Decisions: Cluster Analysis Essay Example
Introduction: Statistics and Research Methodology for Managerial Decisions Assignment - Cluster Analysis
Cluster analysis is the process of grouping similar objects, entities, or people. It is commonly used in research and other tasks to categorize similar groups. Cluster analysis has similarities with factor analysis, especially when applied to individuals (Q-analysis) rather than variables. The first step in cluster analysis involves starting with a homogeneous group and identifying homogenous subgroups based on specific characteristics of the objects.
When measuring similarity, data has been gathered on each of K features for all the N entities under consideration of being divided into groups. Unlike multiple discriminant analysi
...s, cluster analysis does not have predefined groups. The main goal of cluster analysis is to determine the number of distinct groups that exist and define their composition. It analyzes a sample of objects and does not predict relationships.
Terminologies in cluster analysis
- Agglomeration schedule
It refers to a table that indicates the clusters and the objects combined within each cluster, and it can be read from top to bottom.
The tabular array begins with two instances combined and also states the distance coefficients and the first appearance of the phase bunch. The distance coefficients are important in determining the number
of clusters for the data.
- Cluster centroid
This refers to the values of the variable being considered for all instances in a particular cluster. Each cluster will have different centroids for each variable.
- Cluster rank
This is the cluster to which each instance belongs.
To perform ANOVA on the data and determine group importance for analyzing groups, the following are crucial:
- Cluster Center
These are the starting points in non-hierarchical clusters. The clusters are formed around these centers and are therefore referred to as seeds.
- Dendrogram
This is used more frequently in interpreting results than the agglomeration schedule, and it provides an easy way to interpret. The dendrogram is a graphical summary of the cluster solution. The best solution is where the horizontal distance in the graph is maximum.
This might be a subjective process.
- Icicle diagram
It shows how instances are combined into clusters at each analysis loop.
- Similarity/ distance coefficient matrix
This contains the matrix that calculates the distances between the instances.
Measuring methodology
Let theK features be measured byK variables asK1,K2,K3,. . . ,KK.The task of measuring similarity between the objects is complex because, in most cases, the data is measured in different units/scales in its original form. To solve this problem, each variable is
standardized by subtracting its mean value and then dividing by the standard deviation. This converts it into a pure numerical form.
The similarity between two objects, I and J, can be represented as Calciferolij, which is calculated using the formula (teni1 - xj1)2 + (teni2 - xj2)2 + ...... + (tenik- xjk). This calculation indicates the level of similarity between the two objects. To organize groups mathematically, it is important to have a benchmark that can evaluate different groupings and determine the optimal number of objects in each group. The methodology involves using distances among objects from groups to achieve this.
The distance similarity matrix among three objects is displayed as follows:
Distance or similarity matrix
S.no | Oxygen1 | Oxygen2 | Oxygen3 | |
1 | 5 | 2 | 8 | |
2< / td > | 5< / td > | 6< / td > < / tr > | ||
2 | 1 and 3 | 8 | (=6+2) | 16 < /tr > |