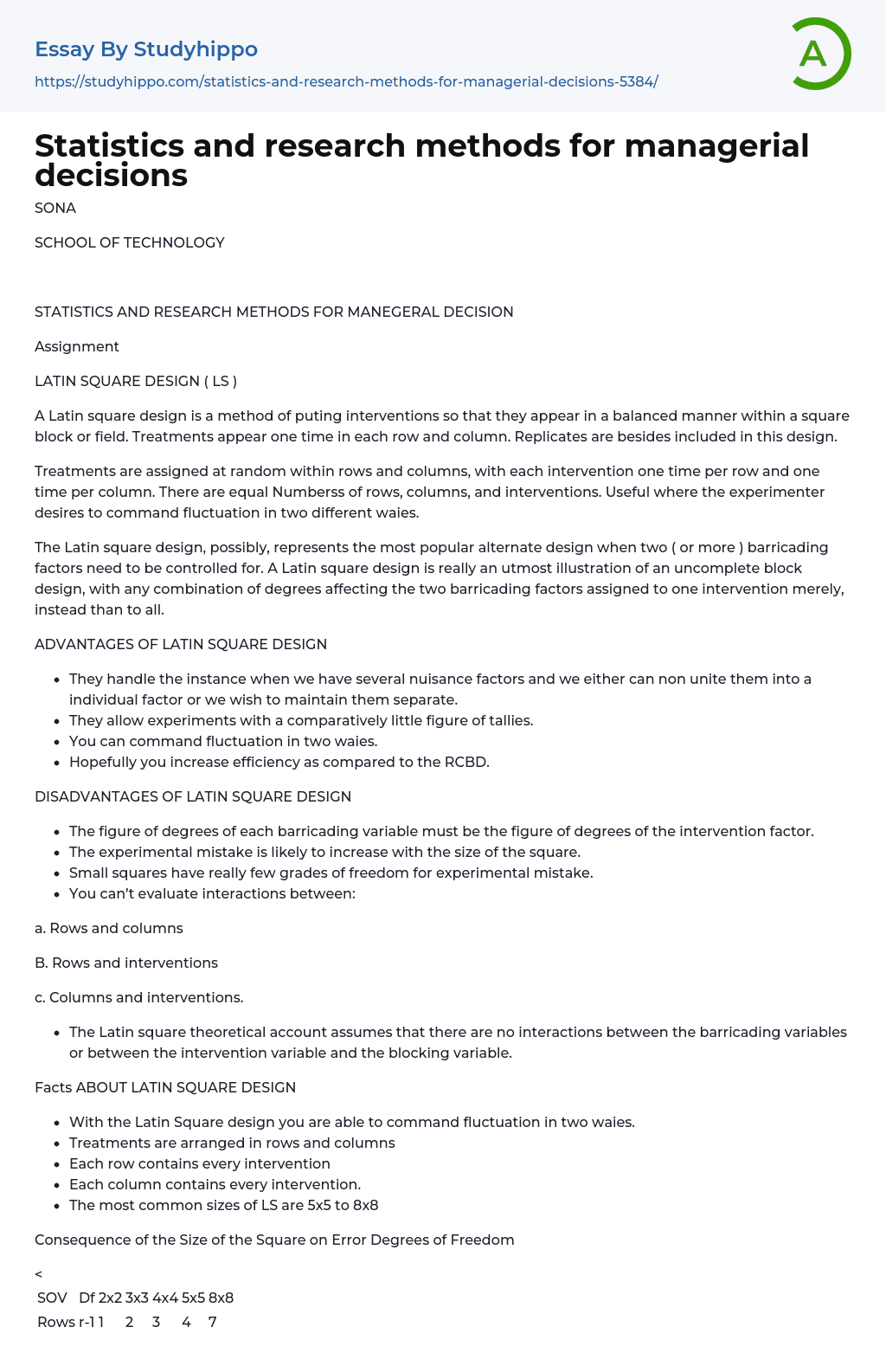
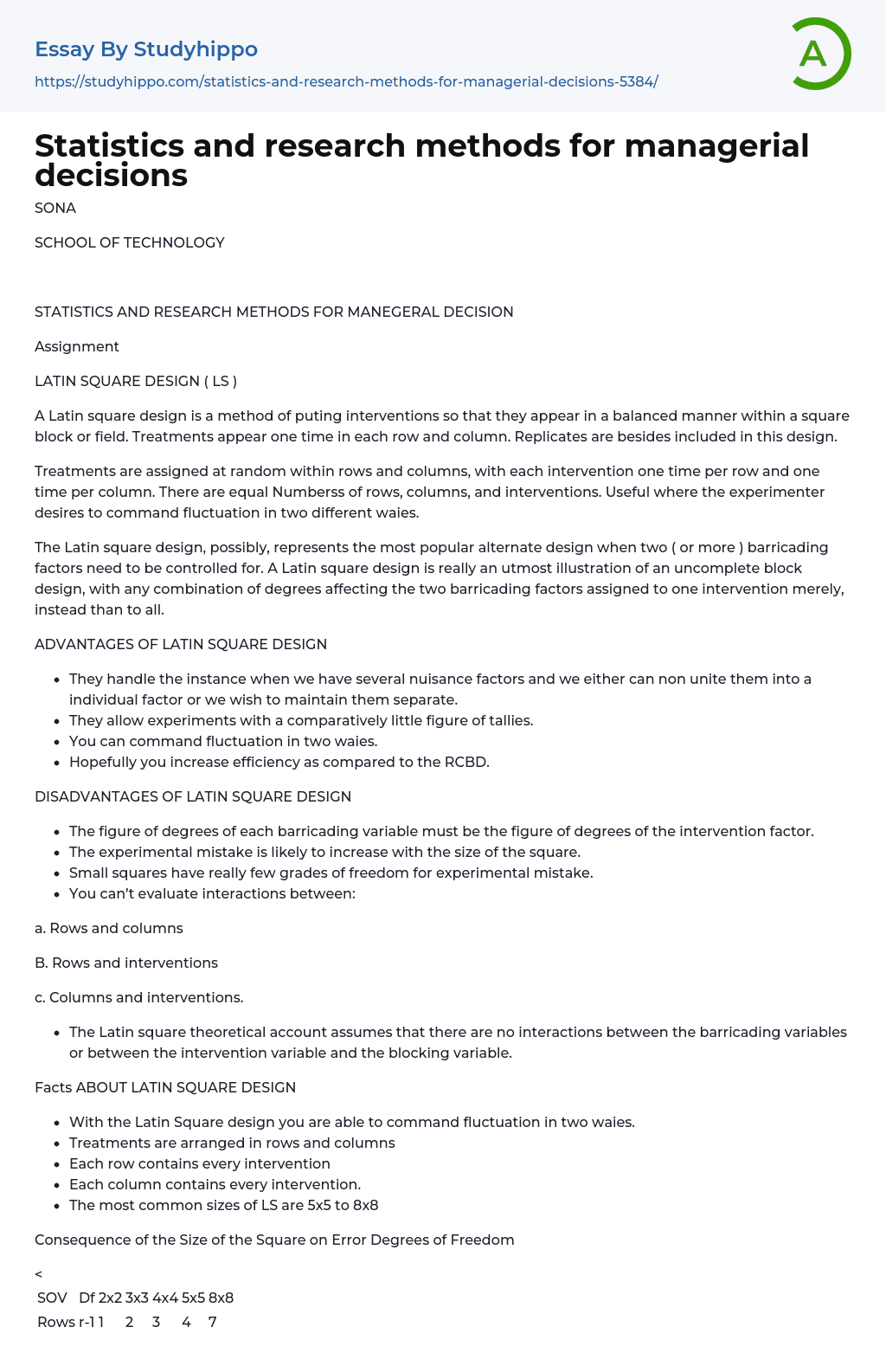
Statistics and research methods for managerial decisions Essay Example
Assignment
LATIN SQUARE DESIGN ( LS )
A Latin square design is a method of putting interventions so that they appear in a balanced manner within a square block or field. Treatments appear one time in each row and column. Replicates are besides included in this design.
Treatments are assigned at random within rows and columns, with each intervention one time per row and one time per column. There are equal Numberss of rows, columns, and interventions. Useful where the experimenter desires to command fluctuation in two different waies.
The Latin square design, possibly, represents the most popular alternate design when two ( or more ) barricading factors need to be controlled for. A Latin square design is really an utmost illustration of an uncomplete block design, with any combination of degrees affecting the two barricading factors assigned to one intervention merely, instead than to all.
ADVANTAGES OF LATIN SQU
...ARE DESIGN
- They handle the instance when we have several nuisance factors and we either can non unite them into a individual factor or we wish to maintain them separate.
- They allow experiments with a comparatively little figure of tallies.
- You can command fluctuation in two waies.
- Hopefully you increase efficiency as compared to the RCBD.
DISADVANTAGES OF LATIN SQUARE DESIGN
- The figure of degrees of each barricading variable must be the figure of degrees of the intervention factor.
- The experimental mistake is likely to increase with the size of the square.
- Small squares have really few grades of freedom for experimental mistake.
- You can’t evaluate interactions between:
- Rows and columns
- Rows and interventions
- Columns and interventions.
- The Latin square theoretical account assumes that there are
no interactions between the barricading variables or between the intervention variable and the blocking variable.
Facts ABOUT LATIN SQUARE DESIGN
- With the Latin Square design you are able to command fluctuation in two waies.
- Treatments are arranged in rows and columns
- Each row contains every intervention
- Each column contains every intervention.
- The most common sizes of LS are 5x5 to 8x8
Consequence of the Size of the Square on Error Degrees of Freedom
SOV | Df | 2x2 | 3x3 | 4x4 | 5x5 | 8x8 |
Rows | r-1 | 1 | 2 | 3 | 4 | 7 |
Column | r-1 | 1 | 2 | 3 | 4 | 7 |
Treatments | r-1 | 1 | 2 | 3 | 4 | 7 |
Mistake | ( r-1 ) ( R-2 ) | 0 | 2 | 6 | 12 | 42 |
Entire | R2- 1 | 3 | 8 | 15 | 24 | 63 |
Where R = figure of rows, columns, and interventions.
- One manner to increase the Error df for little squares is to utilize more than one square in the experiment ( i.e. repeated squares ) .
EXAMPLE-1
Two 4x4 Latin squares.
SOV | Df | |
Squares | sq – 1 = 1 | |
* | Row ( square ) | sq ( r-1 ) = 6 |
* | Column ( square ) | sq ( r-1 ) = 6 |
Treatment | r-1 = 3 | |
Square x Treatment | ( sq-1 ) ( r-1 ) = 3 | |
* | Mistake | sq ( r-1 ) ( r-2 ) = 12 |
Entire | sqr2– 1 = 31 |
*Additive across squares.
Where sq = figure of squares.
Examples of Uses of the Latin Square Design
- Field tests in which the experimental mistake has two birthrate gradients running perpendicular each other or has a unidirectional birthrate gradient but besides has residuary effects from old tests.
Gradient 2
Gradient 1
- Animal scientific discipline provender tests.
- Insecticide field test where the insect migration has a predictable way that is perpendicular to the dominant birthrate gradient of the experimental field.
- Greenhouse tests in which the experimental pots are arranged in a consecutive line perpendicular to the glass walls, such that the difference among rows of pots and distance from the glass wall are
expected to be the major beginnings of variableness.
A D C B | B C A D | D A B C | C B D A |
Randomization Procedure
Depends on the type of Latin Square you use.
3x3 Latin Square
Start with the standard square and randomise all columns and all but the first row.
1 | 1 | 2 | 3 | 3 | 1 | 2 | ||
A | Bacillus | C | Randomize columns | C | A | Bacillus | ||
2 | Bacillus | C | A | A | Bacillus | C | ||
3 | C | A | Bacillus | Bacillus | C | A | ||
Standard square | ||||||||
Randomize all but the first row
C | A | Bacillus |
Bacillus | C | A |
A | Bacillus | C |
4x4 Latin Square
Randomly take a standard square.
Randomize all columns and all but the first row.
5x5 Latin Square
Randomly take a standard Square. Randomize all columns and rows.
Analysis of a Single Latin Square EXAMPLE-2 |
|||||
Grain output of three corns loanblends ( A, B, and D ) and a cheque ( C ) . | |||||
Row | Column 1 | Column 2 | Column 3 | Column 4 | Row ( ?Roentgen) |
1 | 1.640 ( B ) | 1.210 ( D ) | 1.425 ( C ) | 1.345 ( A ) | 5.620 |
2 | 1.475 ( C ) | 1.185 ( A ) | 1.400 ( D ) | 1.290 ( B ) | 5.350 |
3 | 1.670 ( A ) | 0.710 ( C ) | 1.665 ( B ) | 1.180 ( D ) | 5.225 |
4 | 1.565 ( D ) | 1.290 ( B ) | 1.655 ( A ) | 0.660 ( C ) | 5.170 |
Column sum ( ?C) | 6.350 | 4.395 | 6.145 | 4.475 | 21.365 |
STEP-1: Calculate intervention sums.
TreatmentTotal
- 5.855
- 5.885
- 4.270
- 5.355
STEP-2: Calculate the Correction Factor ( CF ) .
Californium=Yttrium..2
R2
- 21.3652
42
- 28.53
STEP-3: Calculate the Total SS
Sum= ?Yttriumij2?Californium
- ( 1.642+1.2102+1.4252+ ... +0.662) ?Californium
- 1.4139
STEP-4: Calculate the Row SS
Row=?RowR2?Californium
=( 5.622+5.352+5.2252+5.172)?Californium4
= 0.0302
Measure 5: Calculate the Column SS.
Col.United states secret service=?ColR2?Californium
=( 6.352+4.3952+6.1452+4.4752)?Californium4
= 0.8273
Measure 6: Calculate the Treatment SS
TrtSS=?RLolo.2?Californium
=( 5.8552+5.8852+ 4.2702+5.3552)?Californium4
src="https://s3-eu-west-1.amazonaws.com/aaimagestore/essays/1423385.010.png" alt="" />
= 0.4268
Measure 7: Calculate the Error SS
Error SS = Total SS – Row SS – Column SS – Trt SS
= 0.129
Measure 8: Complete the ANOVA tabular array
SOV | Df | United states secret service | Multiple sclerosis | F | ||
Row | r-1 = 3 | 0.030 | ||||
Column | r-1 = 3 | 0.827 | Trt MS/Error MS = 6.60* | |||
Trt | r-1 = 3 | 0.427 | 0.142 | |||
Mistake | ( r-1 ) ( r-2 ) = 6 | 0.129 | 0.0215 | |||
Entire | R2-1 = 15 | 1.414 |
STEP- 9: Calculate the LSD.
0.254
MANAGERIAL IMPLICATIONS
The advantage of fewer needed topics is accompanied by terrible disadvantages for usage of the Latin Square experiment design in marketing research. First, interaction effects between independent variables and independent variable values can non be investigated. Therefore, a Latin Square is limited to those state of affairss in which it is sensible to presume that no interaction effects occur, which in the world of a selling determination makes is really improbable.
Second, independent variable values ( i.e. , experimental interventions ) must be equal in figure to the figure of values for each immaterial variable explicitly controlled. Hence, the “square” name of this type of experiment is reflected in the corresponding “square” tabular array that represents its design. However, the existent universe of marketing determination shapers frequently involves state of affairss where the figure of independent variable values varies otherwise than the values of immaterial variables. For illustration, inquiries about gender might affect two values while inquiries about bundle design involve two, three, four, or more values.
Furthermore, these are serious tradeoffs when utilizing a Latin Square experiment for a selling research undertaking. While a positive facet is fewer needed topics, a negative facet is the legion restrictions finding when it can be used. First, it is practical merely with one independent variable. Second, there much ever be an
equal figure of independent variable values and immaterial variable values. Third, there is no trial of interaction effects. In selling, the world is that there is most ever an involvement in more than one independent variable and there are most ever interaction effects between variables. Therefore, despite the positive entreaty of less topics, which means less clip and money, the negative side is a serious restriction as to when this type of experiment can be used in marketing research.
FACTORIAL DESIGNS ( FD )
“A factorial design really consists of a set ofindividual-factor experiments.” That’s why it’s so of import to truly understand the single-factor design! Keppel illustrates the component nature of the factorial design in Table 9-1. Therefore, in the illustration he’s discussing, the length of the printed lines of text could be either 3, 5, or 7 inches. The difference in elation between the print and the paper could besides be manipulated ( low, medium, or high ) . The dependent variable is reading velocity.
3 in. 5 in. 7 in.
Low Contrast
Medium Contrast
High Contrast
We could analyze the simple consequence of line length under Low Contrast. We could besides analyze the simple consequence of line length under Medium Contrast. Finally, we could analyze the simple consequence of line length under High Contrast. In add-on, we could disregard ( norm over ) the assorted degrees of contrast and analyze the chief consequence of line length. Note the existent difference between simple effects and chief effects.
- Note that we could turn the experiment on terminal and think of the 3 simple effects of contrast at changing degrees of line length. We could besides see the chief consequence of contrast
( averaging over all degrees of line length ) .
- Aside from economic system, the existent advantage of factorial designs is the ability to analyze interactions. In kernel, an interaction is present when the simple effects differ. Therefore, if the effects of line length were tantamount regardless of the degree of contrast, we would hold no grounds of an interaction. When an interaction is present, reading of a chief consequence becomes complicated.
The Concept of Interaction
- The chief effects of a factorial design are computed in a manner indistinguishable to the calculation of intervention effects in a individual factor design. ( See, I told you it was truly important to understand the single-factor ANOVA! ) Therefore, the lone new calculation is the interaction term
- Example of an result that illustrates no interaction:
3 in. | 5 in. | 7 in. | Fringy Mean | |
Low Contrast | .89 | 2.22 | 2.89 | 2.00 |
Medium Contrast | 3.89 | 5.22 | 5.89 | 5.00 |
High Contrast | 4.22 | 5.55 | 6.22 | 5.33 |
Fringy Mean | 3.00 | 4.33 | 5.00 | 4.11 |
Why is this result consistent with no interaction? Let’s focal point on the simple effects of line length. ( We could merely as easy have chosen to concentrate on the simple effects of contrast. ) For the Low Contrast status, note that the addition in reading velocity from the 3-inch status to the 5-inch status is 1.33, and the addition from the 5-inch status to the 7-inch status is.67. Although overall reading velocity additions when the contrast is medium, the addition in reading velocity from the 3-inch status to the 5-inch status remains 1.33, and the addition from the 5-inch status to the 7-inch status remains.67. Finally, when the contrast is high, reading velocities show farther betterment, but the addition in reading velocity from the 3-inch status to the 5-inch status remains 1.33, and the addition in
reading velocity from the 5-inch status to the 7-inch status remains.67. The fact that the alterations in reading velocity remain consistent for all the simple effects is declarative of a deficiency of interaction.
- You could besides take a graphical attack to interaction, which is to look for a deficiency of parallel lines in a graph of the status means. As you can seen in the graph below, the lines linking the agencies are parallel.
Average Reading Speed |
Contrast
- In the absence of a important interaction, you would concentrate your attending on the chief effects. That is, because the simple effects are all stating you fundamentally the same narrative, there’s small ground to analyze them individually.
- an illustration of an result that illustrates an interaction:
3 in. | 5 in. | 7 in. | Fringy Mean | |
Low Contrast | 1.00 | 2.00 | 3.00 | 2.00 |
Medium Contrast | 3.00 | 5.00 | 7.00 | 5.00 |
High Contrast | 5.00 | 6.00 | 5.00 | 5.33 |
Fringy Mean | 3.00 | 4.33 | 5.00 | 4.11 |
( Note that the fringy agencies remain the same as those in the illustration demoing no interaction. Therefore, the chief effects are indistinguishable. )
- In this illustration, you can rapidly see that the simple effects are non the same. This result is peculiarly apparent when looking at a figure of the information. ( Note the non-parallel lines. )
Average Reading Speed |
Like Figure 9-3
8
6
4 | ||||
7 in. | ||||
2 | 5 in. | |||
3 in. | ||||
0 | ||||
Low | Medium | High | ||
Contrast
- When an interaction is present, we are most frequently less interested in the chief effects. Alternatively, we typically focus on interpreting/understanding the interaction.
- It provides a figure of definitions of an interaction. In instance any one of them may assist you to understand the construct, I’ll merely copy them for you here:
An interaction is present when the effects of one independent variable on behavior alteration at the different degrees of the 2nd independent variable. ( To maintain in head that the
two independent variables do non act upon one another! )
An interaction is present when the form of differences associated with an independent variable alterations at the different degrees of the other independent variable.
An interaction is present when the simple effects of one independent variable are non the same at all degrees of the 2nd independent variable.
An interaction is present when the chief consequence of an independent variable is non representative of the simple effects of that variable.
An interaction is present when the differences between cell means stand foring the effects of Factor A at one degree of Factor B do non be the corresponding differences at another degree of Factor B.
An interaction is present when the effects of one of the independent variables are conditionally related to the degrees of the other independent variable.
An interaction is present when one of the independent variables does non hold a changeless consequence at all degrees of the other independent variable.
- Although the presence of an interaction may perplex the explication of the consequences of an experiment, you should recognize that many experiments really predict an interaction. Therefore, you may good be looking to happen an interaction in an experiment.
- It provides a figure of 2x2 results to exemplify the presence or absence of an interaction. You should be certain that you can easy find that an interaction appears to be present or absent in an experiment. Of class, the concluding supreme authority of the presence of an interaction is non a graph, but the statistical trial that you will happen in the beginning tabular array. Even though lines may look to be non-parallel, if the F for the
interaction is little ( i.e. , the P value is above.05 ) , so the lines are statistically parallel and there is no important interaction.
Mentions:
- Books referred:
- Designs and methods by Mr. C K Kothari.
- Research Methodology by Mr. Paneersalvem
- Business Research and Methodology by Mr. Srivastava and Mr. Rego.
- Normal Distribution essays
- Probability Theory essays
- Variance essays
- Accident essays
- Awareness essays
- Benefits of Volunteering essays
- Challenges essays
- Childhood Memories essays
- Decision essays
- Driving essays
- Event essays
- Excellence essays
- Expectations essays
- Failure essays
- Farewell essays
- Flight essays
- Gift essays
- Growing Up essays
- Ignorance essays
- Improve essays
- Incident essays
- Knowledge essays
- Luck essays
- Memories essays
- Mistake essays
- Obstacles essays
- Overcoming Challenges essays
- Party essays
- Peace Corps essays
- Personal Experience essays
- Problems essays
- Sacrifices essays
- Struggle essays
- Success essays
- Trust essays
- Vacation essays
- Visit essays
- Volunteering essays
- Experiment essays
- Explorer essays
- Hypothesis essays
- Observation essays
- Qualitative Research essays
- Research Methods essays
- Theory essays
- Algebra essays
- Arithmetic essays
- Correlation essays
- Geometry essays
- Measurement essays