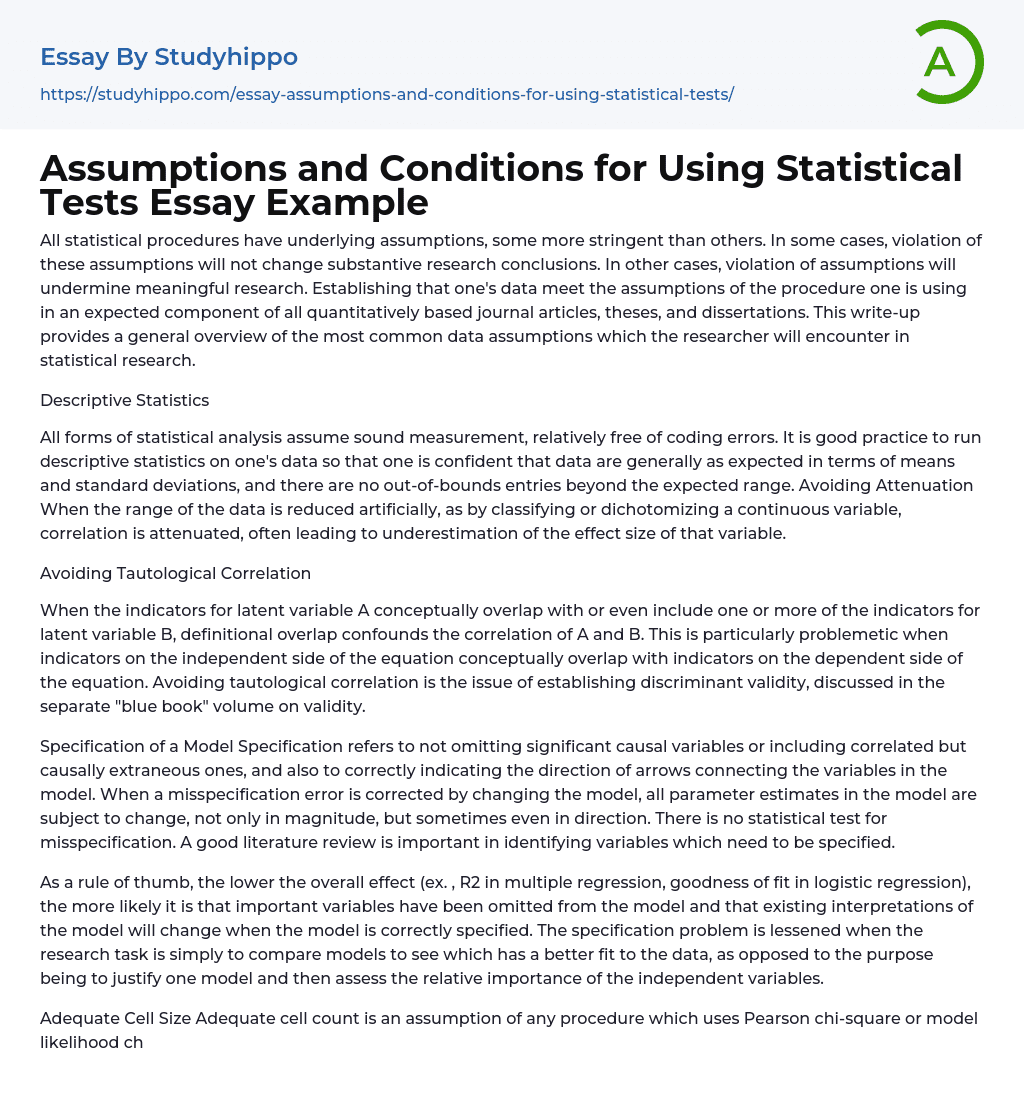
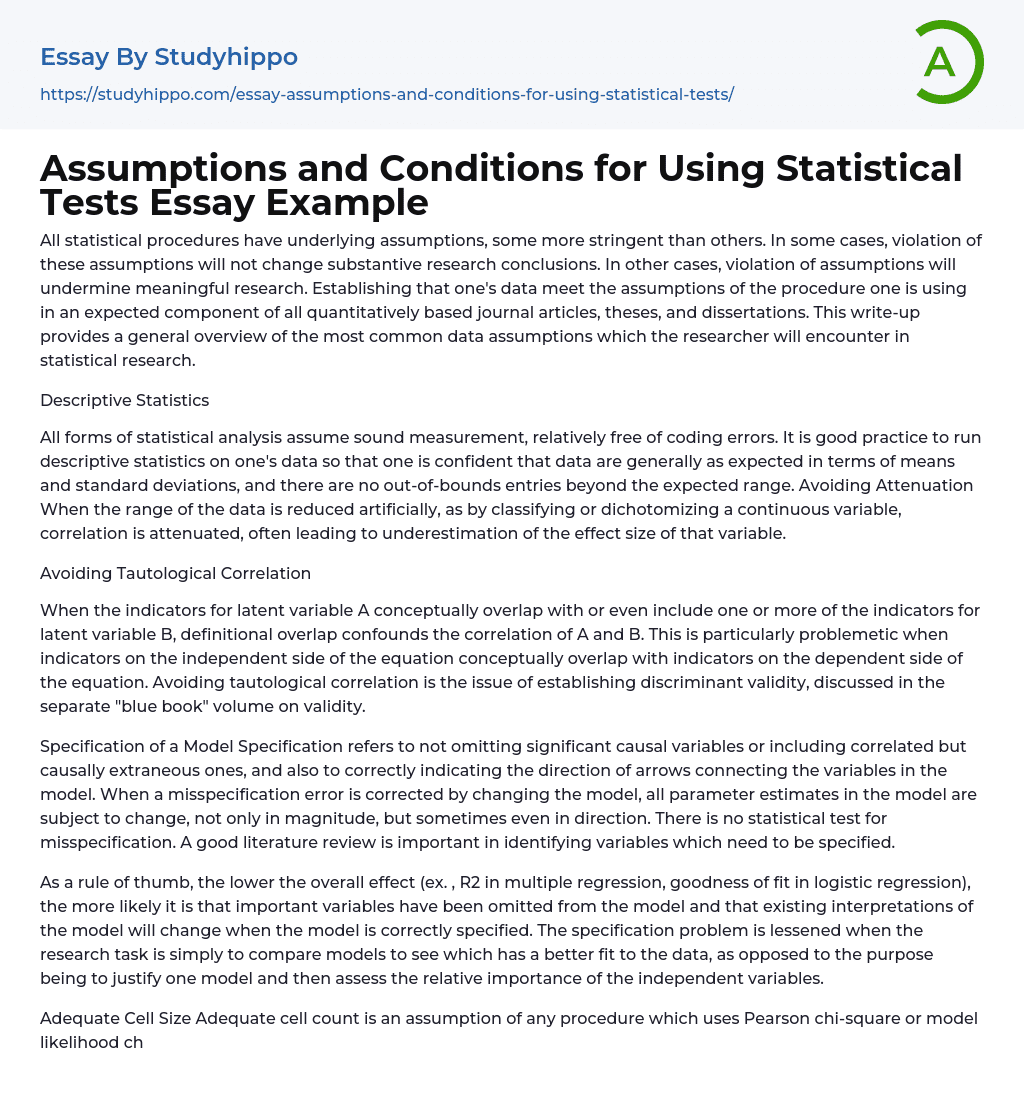
Assumptions and Conditions for Using Statistical Tests Essay Example
All statistical procedures have underlying assumptions, some more stringent than others. In some cases, violation of these assumptions will not change substantive research conclusions. In other cases, violation of assumptions will undermine meaningful research. Establishing that one's data meet the assumptions of the procedure one is using in an expected component of all quantitatively based journal articles, theses, and dissertations. This write-up provides a general overview of the most common data assumptions which the researcher will encounter in statistical research.
Descriptive Statistics
All forms of statistical analysis assume sound measurement, relatively free of coding errors. It is good practice to run descriptive statistics on one's data so that one is confident that data are generally as expected in terms of means and standard deviations, and there are no out-of-bounds entries beyond the expected range. Avoi
...ding Attenuation When the range of the data is reduced artificially, as by classifying or dichotomizing a continuous variable, correlation is attenuated, often leading to underestimation of the effect size of that variable.
Avoiding Tautological Correlation
When the indicators for latent variable A conceptually overlap with or even include one or more of the indicators for latent variable B, definitional overlap confounds the correlation of A and B. This is particularly problemetic when indicators on the independent side of the equation conceptually overlap with indicators on the dependent side of the equation. Avoiding tautological correlation is the issue of establishing discriminant validity, discussed in the separate "blue book" volume on validity.
Specification of a Model Specification refers to not omitting significant causal variables or including correlated but causally extraneous ones, and also to correctly
indicating the direction of arrows connecting the variables in the model. When a misspecification error is corrected by changing the model, all parameter estimates in the model are subject to change, not only in magnitude, but sometimes even in direction. There is no statistical test for misspecification. A good literature review is important in identifying variables which need to be specified.
As a rule of thumb, the lower the overall effect (ex. , R2 in multiple regression, goodness of fit in logistic regression), the more likely it is that important variables have been omitted from the model and that existing interpretations of the model will change when the model is correctly specified. The specification problem is lessened when the research task is simply to compare models to see which has a better fit to the data, as opposed to the purpose being to justify one model and then assess the relative importance of the independent variables.
Adequate Cell Size Adequate cell count is an assumption of any procedure which uses Pearson chi-square or model likelihood chi-square (deviance chi-square) in significance testing when categorical predictors are present. This includes but is not limited to chi-square testing of crosstabulation, loglinear analysis, binomial logistic regression, multinomial logistic regression, ordinal regression, and general or generalized linear models of the same.
Factor Space
Factor space is the set of cells which are generated by a crosstabulation of the categorical dependent with all the categorical factors but not the continuous covariates.
Data Level Requirements Measurement level requirements vary by statistical procedure but most procedures require an interval or ratio level of measurement. It is common in
social science, however, to utilize dichotomies and ordinal data, such as Likert scale data, even in procedures which technically require interval-level data.
Dichotomies are often included in statistical models (ex. , regression models) provided the split is less than 90:10. Ordinal variables are often included in statistical models provided the normality of error terms may be demonstrated, as discussed below. Some researchers require the ordinal scale to have five or more values. Violations of data level assumptions mean that actual standard error will be greater than the computed standard error and significance is overestimated (that is, the chance of Type I error is greater than computed).
- Research Methods essays
- Experiment essays
- Hypothesis essays
- Observation essays
- Qualitative Research essays
- Theory essays
- Explorer essays
- Normal Distribution essays
- Probability Theory essays
- Variance essays
- Algebra essays
- Arithmetic essays
- Correlation essays
- Geometry essays
- Measurement essays
- Price Elasticity Of Demand essays
- Regression Analysis essays
- Statistics essays
- Agriculture essays
- Albert einstein essays
- Animals essays
- Archaeology essays
- Bear essays
- Biology essays
- Birds essays
- Butterfly essays
- Cat essays
- Charles Darwin essays
- Chemistry essays
- Dinosaur essays
- Discovery essays
- Dolphin essays
- Elephant essays
- Eli Whitney essays
- Environmental Science essays
- Evolution essays
- Fish essays
- Genetics essays
- Horse essays
- Human Evolution essays
- Isaac Newton essays
- Journal essays
- Linguistics essays
- Lion essays
- Logic essays
- Mars essays
- Methodology essays
- Mineralogy essays
- Monkey essays
- Moon essays