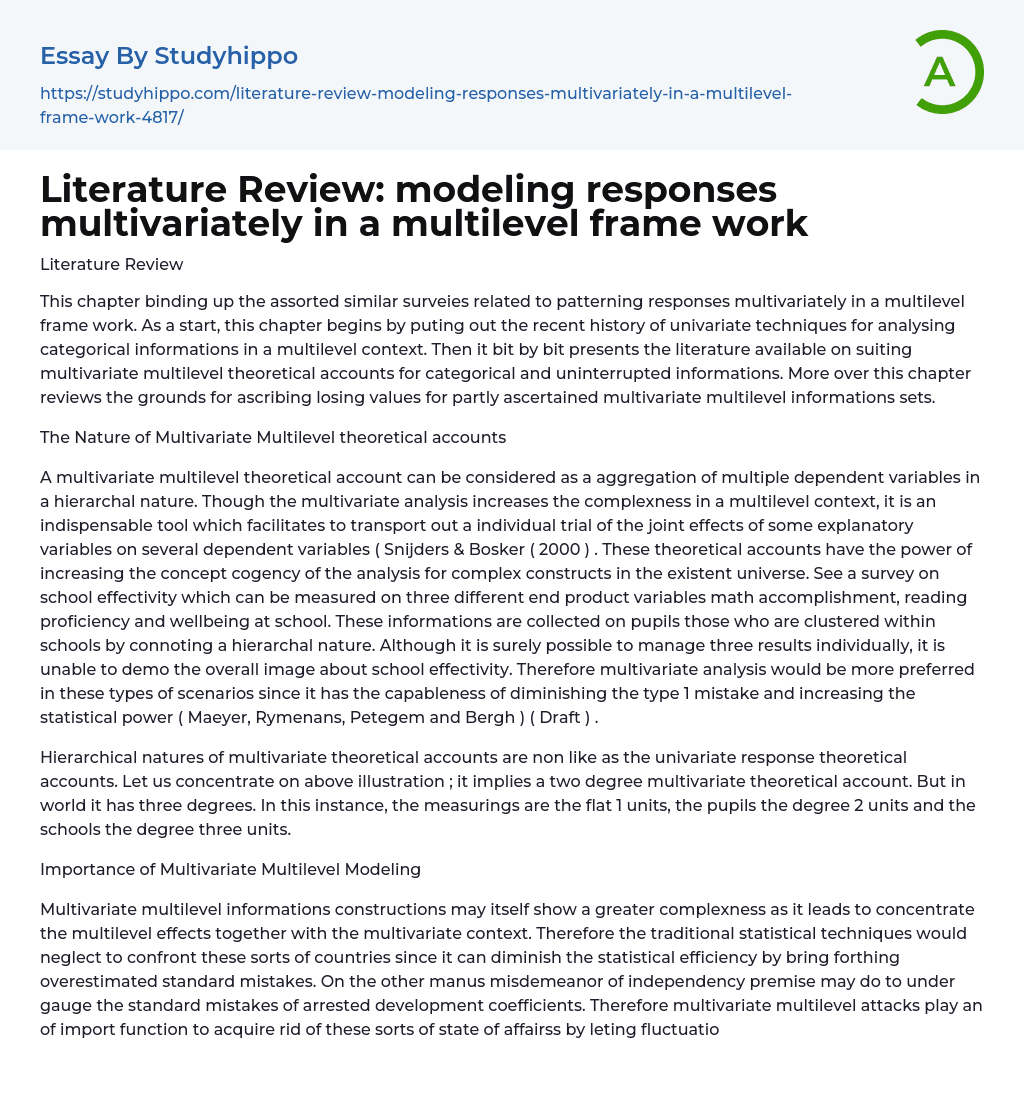
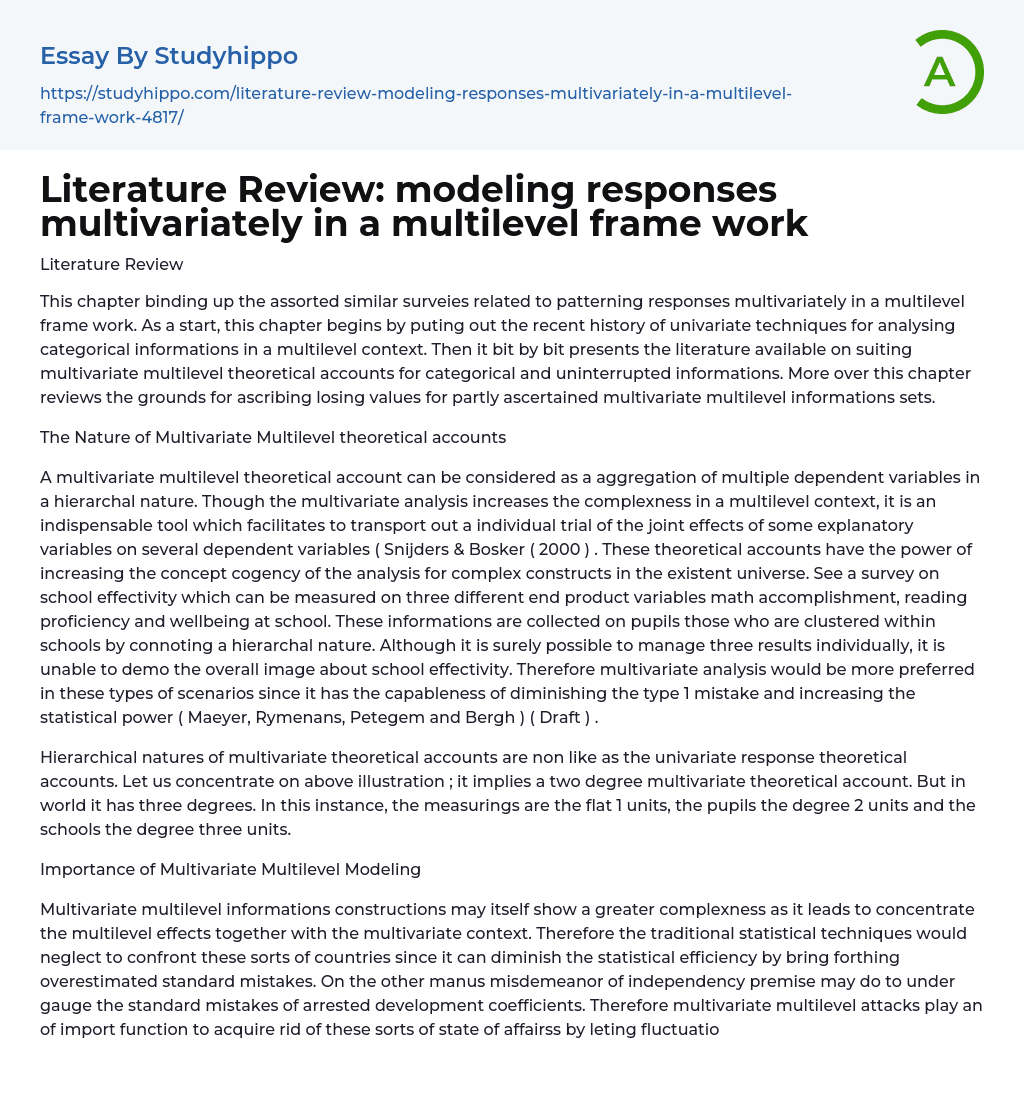
Literature Review: modeling responses multivariately in a multilevel frame work Essay Example
Literature Review
This section contains a compilation of various studies that are relevant to modeling multivariate responses in a multilevel framework.
The text examines the recent history of analyzing categorical information in a multilevel context using univariate techniques. It also explores the literature on adapting multivariate multilevel models for both categorical and continuous information and discusses why missing values are assigned in partially ascertained multivariate multilevel data sets.
The Nature of Multivariate Multilevel Models
A multivariate multilevel model is a hierarchical structure with multiple dependent variables. Although it adds complexity to the analysis in a multilevel context, it allows for testing the combined effects of explanatory variables on multiple dependent variables simultaneously (Snijders & Bosker, 2000). These models enhance the validity of analyzing complex concepts in real-world scenarios.
The survey investigates the effectiveness of schools by a
...ssessing three variables: math achievement, reading proficiency, and school well-being. The data is gathered from students who are organized in a hierarchical manner within schools. Examining each variable individually does not provide a comprehensive understanding of school effectiveness. Therefore, it is suggested to utilize multivariate analysis in these situations as it can decrease type 1 errors and enhance statistical power. Multivariate analysis distinguishes itself from univariate response models due to the hierarchical structure of the variables.
The above illustration depicts a theoretical model with two degrees, however in actuality, it consists of three degrees. In this instance, the measurements are represented by level 1 units, the pupils by level 2 units, and the schools by level 3 units.
The Significance of Multivariate Multilevel Modeling
Multivariate multilevel data structures can be more complex as they encompass both multilevel effects and the multivariate context. Conventional statistica
techniques may not effectively handle these scenarios and could lead to increased standard errors, thus reducing statistical efficiency.
The use of multivariate multilevel approaches is crucial for addressing the underestimation of development coefficients due to violations of independence assumptions. This approach allows for estimating variation at different levels and improves accuracy in standard errors, confidence intervals, and significance tests (Goldstein, 1999). While there have been numerous articles published on multilevel modeling focused on a single response context, the concept of multivariate multilevel has recently emerged in statistics. When examining the effects of a set of explanatory variables on a set of dependent variables, conducting multivariate analysis becomes necessary if there is a significant difference among these effects (Snijders & Bosker, 2000).
Software for Multivariate Multilevel Modeling
Previously, researchers encountered difficulties in finding appropriate software to handle multivariate multilevel data. This led them to resort to manual methods like the EM Algorithm (Kang et al., 1991). However, there are now statistical software packages available, such as STATA, SAS, and S plus, that can assist with managing multilevel data. Unfortunately, none of these packages have the capability to handle multivariate multilevel data. Existing literature suggests that GLLAMM (Rabe-Hesketh, Pickles, and Skrondal, 2001) and aML (Lillard and Panis, 2000) can be used for fitting nonlinear multivariate multilevel models. However, these packages were deemed insufficiently flexible for meeting all requirements.
The University of Bristol in the UK has been developing a modified version of the MlwiN package since the late 1980's. In response to demand, modifications were made at the same university. However, Goldstein, Carpenter, and Browne (2014) challenged the use of MlwiN for multivariate multilevel theoretical models. They concluded that MlwiN
is only useful for fitting the model if missing values are not taken into account. To address this limitation, the REALCOM package was introduced in Statistics. It allows for flexibility in accounting for missing values within the MLwiN environment. MLwiN is based on a command-driven interface and is a modified version of the DOS MLn program.
MLwiN provides a user-friendly interface that allows for flexible modeling of large and complex theoretical models. It supports both frequentist and Bayesian estimation methods, as well as missing value imputation. Additionally, MLwiN offers unique advanced features not found in other software.
Difference between Univariate Multilevel Modeling and Multivariate Multilevel Modeling
In general, when collecting data on multiple outcomes that are correlated with each other, the challenge lies in modeling the relationship between risk factors and each outcome individually.
The statistical efficiency of ignoring outcome correlativities and common forecaster effects (Oman, Kamal and Ambler) may be questionable (unpublished). Therefore, instead of using univariate models, many researchers opt to include all related results in an individual arrested development theoretical account within a multivariate result model. Recent studies have compared Univariate and Multivariate results and have shown that Multivariate models are preferable over several univariate models. In a study conducted by Griffiths, Brown, and Smith (2004), they compared univariate and multivariate multilevel models for recurrent measures of usage of prenatal care in Uttar Pradesh, India. They examined various factors that may influence a mother's decision to utilize ante-natal care services for a specific pregnancy. The study compared Univariate multilevel logistic arrested development theoretical model vs.
Multivariate multilevel logistic arrested development theoretical account was chosen over univariate theoretical accounts due to the violation of model premises and instability
in parameter estimations. Therefore, the analysis was conducted in a multivariate context.
Generalized Cochran Mantel Haenzel Tests for Checking Association of Multilevel Categorical Data
The origins of Generalized Cochran Mantel Haenzel can be traced back to the late 1950's. Cochran (1958), a renowned Statistician, initially proposed a test to determine the independence of multiple 2 ? 2 tables by extending the general chi-square test for independence of a single 2-way table.
The tabular arrays contain additional variables to account for higher-degree observations, allowing for the observation of the multilevel nature. The trial statistic is calculated based on the row sums of each tabular array. The underlying assumption is that the cell counts follow a binomial distribution. Building upon Cochran's work, Mantel and Haenzel (1959) expanded the trial statistic to include both row and column sums, assuming that the cell counts in each tabular array follow a hypergeometric distribution.
Due to a major limitation in binary data, Cochran Mantel Hanzel (CMH) statistic was extended by Landis et al. (1978) to encompass more than two degrees. However, the Generalized Cochran Mantel Haenzel (GCMH) trial had a significant drawback in managing clustered correlative categorical data. In an attempt to address this issue, Liang (1985) proposed a trial statistic but encountered major challenges and was ultimately unsuccessful in utilizing it.
The development of the statistics field has created a demand for a trial statistic that can handle correlated information and variables with higher degrees. Zhang and Boos (1995) introduced three trial statistics, Elevation, Thymine Phosphorus, and Uracil, as a solution to this issue. However, when comparing these three trial statistics, Phosphorus and Uracil are preferred over Elevation. This preference is due to Phosphorus and
Uracil using single topics as the primary sampling units, while Elevation uses strata as the primary sampling unit (De Silva and Sooriyarachchi, 2012). Additionally, through a simulation survey, it was found that Phosphorus performs better than Elevation. Phosphorus maintains its error values even when the strata are small and it uses pooled calculators for discrepancy. Therefore, Phosphorus is recommended as the most suitable statistic for conducting this survey.
Missing Value Imputation in Multivariate Multilevel Framework
De Silva and Sooriyarachchi ( 2012 ) developed a R plan to carry out this trial. The issue of dealing with missing values is often found in real-world datasets. However, these datasets provide little or no information about the missing data mechanism (MDM). Consequently, modeling incomplete data is a challenging task and may produce biased results. This major problem highlights the need for an appropriate mechanism to examine the missingness.
As a solution to the issue, Rubin (1976) suggests three ways in which missingness can occur: Missing At Random (MAR), Missing Wholly At Random (MCAR), and Missing Not At Random (MNAR). According to Sterne et al. (2009), imputation of missing values is required assuming missingness at random, but it can also be performed assuming missingness completely at random. Currently, most statistical packages can identify the type of missingness.
After identifying the type of missingness, the next step is to use losing value imputation. This requires a statistical package to be used. However, losing value imputation in a hierarchical nature is more complex and cannot be done using standard statistical packages like SPSS, SAS, and R. To address this issue, Carpenter et al. (2009) developed the REALCOM package specifically for
this task. However, the earlier version of REALCOM did not handle multilevel data in a multivariate context. As a solution, the Bristol University team recently developed macros to perform this task.
Estimation Procedure
The estimation process for multilevel mold began in the late 1980s. Early statisticians used the EM algorithm, an iterative procedure, to perform parameter estimation using Maximum Likelihood Method (Raudenbush, Rowan, and Kang, 1991). The HLM program was later developed to execute this algorithm. For estimating multivariate multilevel models with Normal responses, the most effective procedures are Iterative Generalized Least Squares (IGLS), Reweighted IGLS (RIGLS), and Marginal Quasi Likelihood (MQL). For distinct responses, MQL and Penalized Quasi Likelihood (PQL) are used. According to Rasbash, Steele, Browne, and Goldstein (2004), all of these methods, including first or second-order Taylor Series expansions, are implemented in MLwiN. However, it is worth noting that these likelihood-based frequentist methods tend to overestimate precision.
The use of Marcov Chain Monte Carlo (MCMC) methods in implementing Bayesian models has been employed for parameter estimation. These MCMC estimations, performed in MLwiN, yield consistent results but require a large number of simulations to control highly correlated chains. Previous research has also focused on univariate and multivariate multilevel models, specifically studying univariate multilevel logit models. Before diving into the literature on multivariate multilevel analysis, it is important to consider the literature on univariate multilevel analysis since this thesis incorporates some univariate multilevel models before integrating the multivariate ones. Multilevel models for binary data have been widely used by social scientists over the past decades.
In order to properly assess their work with less technology, it is crucial to review their implementation. Guo and Zhao (2000) conducted a
review of the methodologies, hypothesis testing, and hierarchical nature of the data in past literature. Additionally, they provided two examples to support their findings. Firstly, they compared estimates obtained from MQL and PQL methods using MLn and the GLIMMIX method implemented by SAS. By using these examples, they demonstrated that the differences in PQL 1 and PQL 2 are minimal when fitting binary logistic models.
In addition, it has been demonstrated that PQL-1, PQL-2, and GLIMMIX are likely to be suitable for most prior studies in the field of social sciences. Noortgate, Boeck, and Meulders (2003) utilized multilevel binary logit models to analyze Item Response Theory (IRT) models. In order to accomplish this, they evaluated the nine achievement scores for reading comprehension among primary school students in Belgium. They conducted a multilevel analysis using cross-classified logistic multilevel models and employed the GLIMMIX macro from SAS, as well as the MLwiN package.
However, due to convergence issues with PQL methods in MLwiN, SAS was used for analysis instead. Furthermore, it has been demonstrated that the cross-division multilevel logistic model can effectively handle IRT data and estimate parameters even when dealing with imbalanced data.
- Multivariate Multilevel Models
In recent years, there have been limited attempts to apply multivariate multilevel models in practical situations. The majority of these studies have concentrated on educational and socioeconomic domains.
The main focus of their concentration was not on medical scenarios, as none of them were able to do so. However, this chapter discusses the lack of multivariate multilevel analysis in the field of wellness and medical scientific disciplines. It includes literature on multivariate multilevel theoretical models from other
fields.
In previous education studies, Xin Ma (2001) investigated the relationship between academic achievements and student backgrounds in Canada. He considered three levels of involvement and developed a three-level Hierarchical Linear Model (HLM) to achieve his goals. This research allowed him to conclude that both students and schools achieved different levels of success in various subject areas, with a more noticeable disparity among students than among schools. However, the validity of this study depends on certain assumptions about students' prior cognitive abilities.
The study conducted in Chicago by Raudenbush, Johnson, and Sampson (2003) focused on criminal behavior at the individual and neighborhood levels. They used a Rasch model with random effects to analyze the data, assuming conditional independence and additives. Similarly, Yang et al. (2002) examined exam results using a multivariate multilevel analysis. Their study utilized data from two math exams in England in 1997 and considered student characteristics at both the individual and institutional levels. Initially, they started with a simpler model of multivariate normality without incorporating random effects at the institutional level. However, they gradually increased complexity by including institutional levels and multivariate responses.
When examining the work closely, it can be seen that the choice of topic greatly influences the performance. In addition to the increased use of multivariate multilevel models in various fields, researchers can also apply them to areas such as Forestry. Hall and Clutter (2004) conducted a study on the growth and yield patterns in forestry based on cut pine trees in the U.S.A. In their research, they developed a methodology that incorporates a nonlinear mixed effects model within a multivariate multilevel framework to identify the effects of different plot-level lumber measurement
characteristics on lumber volume output.
In addition, they also developed a methodology to generate predictions and prediction intervals from those models. Using their developments, they predicted lumber growth and output at the plot level and population level. Grilli and Rampichini (2003) conducted a study to model ordinal response variables based on the students' evaluation data obtained from a survey of course quality conducted by the University of Florence in the 2000-2001 academic years. For that, they developed an alternative specification to the multivariate multilevel probit ordinal response models by considering responses as an additional unobserved level variable. However, they have not yet evaluated the efficiency of that method since they have not implemented it using standard software. When considering the evidence from recent applications of these models, the literature shows that Goldstein and Kounali (2009) recently conducted a study on child growth with respect to the collection of growth measurements and adult features.
The authors extended the latent normal theoretical account for multilevel informations with assorted response types to ordinal categorical responses with multiple classs for covariates. Instead of assuming a Poison distribution, they treated the counts as ordered classs. In 2007, Frank, Cerda, and Rendon conducted a study to determine the impact of residential location on the health risk behaviors of Latino immigrants. They used a Multivariate Multilevel Rasch theoretical model to analyze data obtained from the Los Angelis household and neighborhood survey, which included indices of health risk behaviors and drug use, as well as participation in risk-based activities.
They begin this effort by studying the behavior of teenagers to understand the factors related to both individuals and communities. A study revealed a connection between increased
health risk behaviors and above-average levels of poverty and Latinos, particularly among those born in the USA. Another study carried out by Subramanian, Kim, and Kawachi (2005) in the USA aimed to identify the factors at both individual and community levels that contribute to individuals' health and happiness. They conducted a multivariate multilevel regression analysis using data from a survey conducted in 2000. The results showed a strong correlation between poor health and unhappiness with individual-level characteristics. Although there have been some studies conducted on education and social sciences in other countries, there is a lack of research focused on health and medical sciences.
Consequently, conducting a survey to analyze global mortality rates of various violent diseases is crucial. This will help gain insights into the risk factors and patterns associated with these diseases, providing the general public and policy makers with valuable information.
- Research Methods essays
- Experiment essays
- Hypothesis essays
- Observation essays
- Qualitative Research essays
- Theory essays
- Explorer essays
- Normal Distribution essays
- Probability Theory essays
- Variance essays
- Cloud Computing essays
- Computer Science essays
- Consumer Electronics essays
- Data Analysis essays
- Electronics essays
- engineering essays
- Enterprise Technology essays
- Hardware essays
- Impact of Technology essays
- Information Age essays
- Information Technology essays
- Modern Technology essays
- Operating Systems essays
- people search essays
- Robot essays
- American Literature essays
- Between The World and Me essays
- Book Report essays
- Book Review essays
- Book Summary essays
- Books essays
- Character essays
- Coming of Age essays
- Dante's Inferno essays
- Everyday Use essays
- Flowers for Algernon essays
- Genre essays
- Greek Mythology essays
- Incidents in The Life of a Slave Girl essays
- Letter essays
- Literary Criticism essays
- Literary devices essays
- Literature Review essays
- Metaphor essays
- Myth essays
- Play essays
- Plot essays
- Poem essays
- Poetry Analysis essays
- Protagonist essays