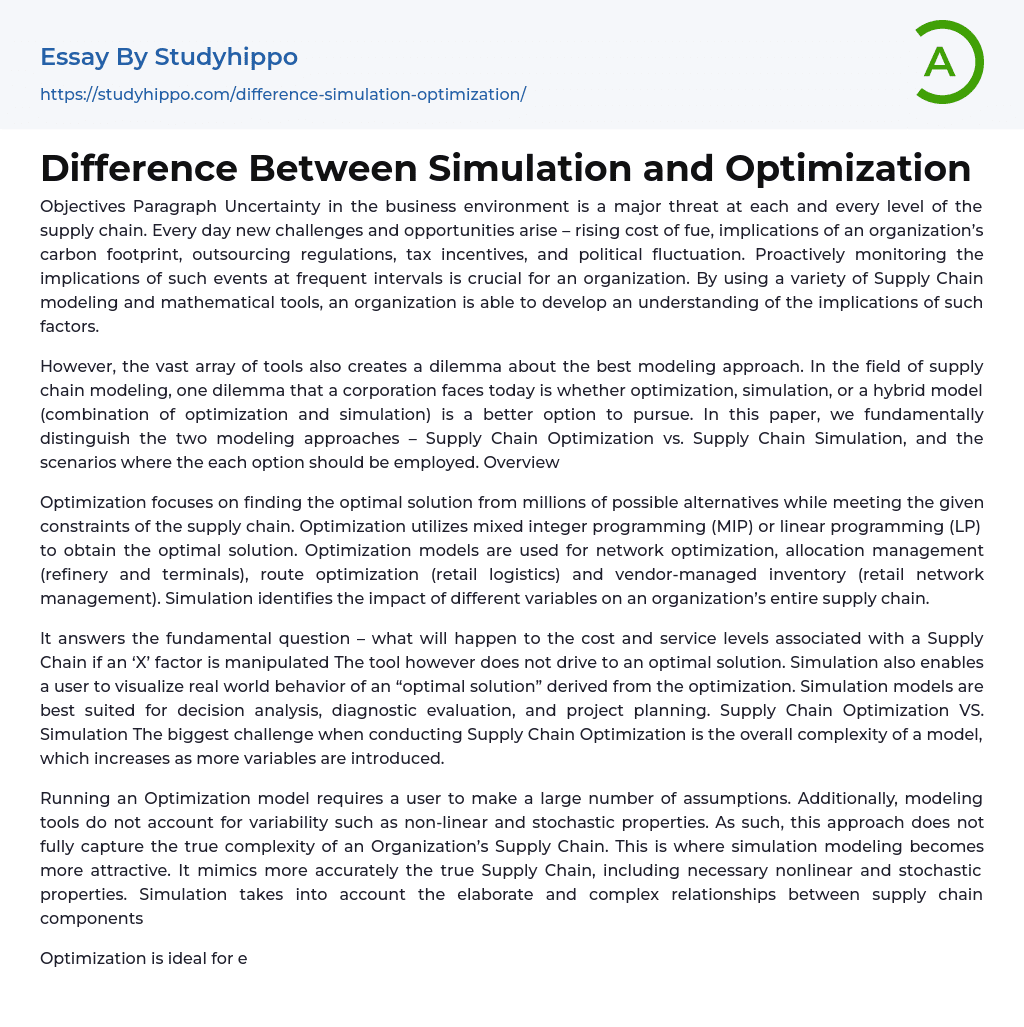
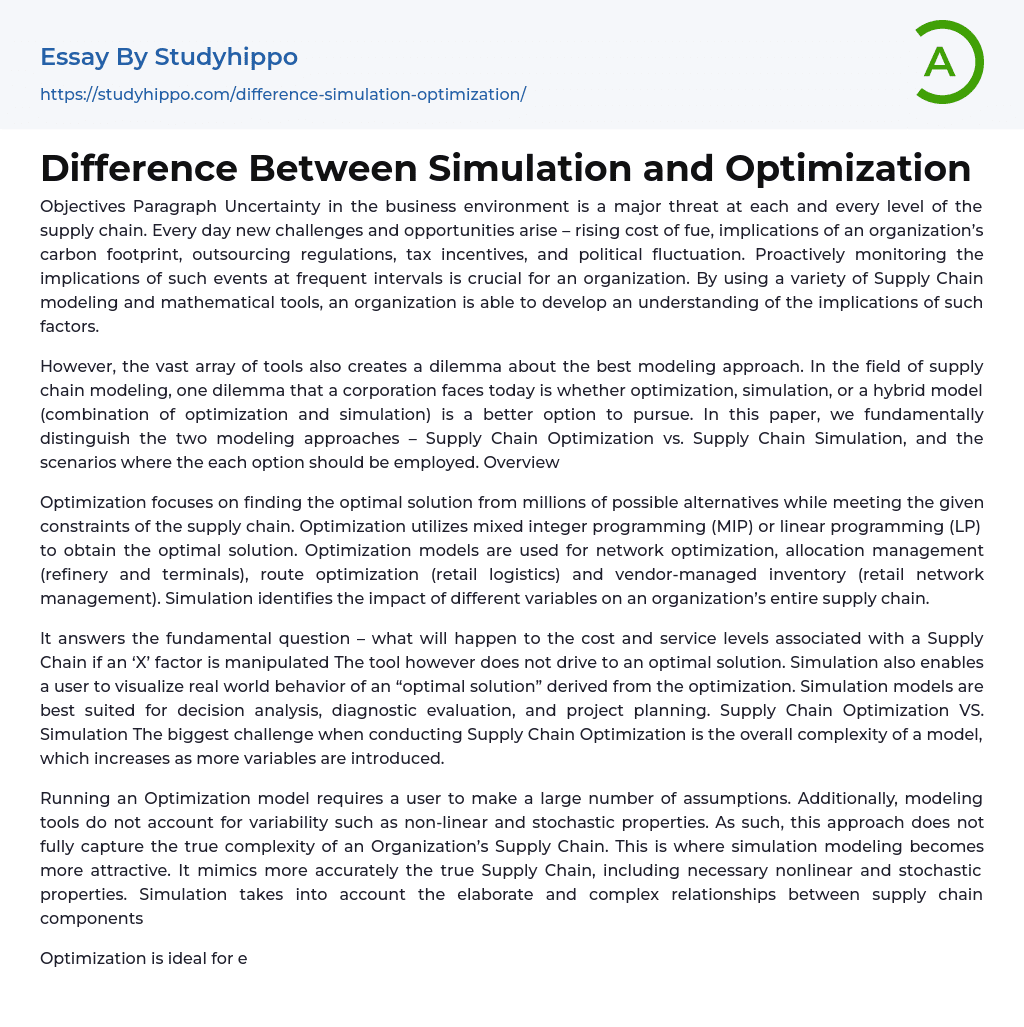
Difference Between Simulation and Optimization Essay Example
Objectives Paragraph Uncertainty in the business environment is a major threat at each and every level of the supply chain. Every day new challenges and opportunities arise – rising cost of fue, implications of an organization’s carbon footprint, outsourcing regulations, tax incentives, and political fluctuation. Proactively monitoring the implications of such events at frequent intervals is crucial for an organization. By using a variety of Supply Chain modeling and mathematical tools, an organization is able to develop an understanding of the implications of such factors.
However, the vast array of tools also creates a dilemma about the best modeling approach. In the field of supply chain modeling, one dilemma that a corporation faces today is whether optimization, simulation, or a hybrid model (combination of optimization and simulation) is a better option to pursue. In this paper, we fundamentally distinguish the two modeling
...approaches – Supply Chain Optimization vs. Supply Chain Simulation, and the scenarios where the each option should be employed. Overview
Optimization focuses on finding the optimal solution from millions of possible alternatives while meeting the given constraints of the supply chain. Optimization utilizes mixed integer programming (MIP) or linear programming (LP) to obtain the optimal solution. Optimization models are used for network optimization, allocation management (refinery and terminals), route optimization (retail logistics) and vendor-managed inventory (retail network management). Simulation identifies the impact of different variables on an organization’s entire supply chain.
It answers the fundamental question – what will happen to the cost and service levels associated with a Supply Chain if an ‘X’ factor is manipulated The tool however does not drive to an optimal solution. Simulation also enables a user
to visualize real world behavior of an “optimal solution” derived from the optimization. Simulation models are best suited for decision analysis, diagnostic evaluation, and project planning. Supply Chain Optimization VS. Simulation The biggest challenge when conducting Supply Chain Optimization is the overall complexity of a model, which increases as more variables are introduced.
Running an Optimization model requires a user to make a large number of assumptions. Additionally, modeling tools do not account for variability such as non-linear and stochastic properties. As such, this approach does not fully capture the true complexity of an Organization’s Supply Chain. This is where simulation modeling becomes more attractive. It mimics more accurately the true Supply Chain, including necessary nonlinear and stochastic properties. Simulation takes into account the elaborate and complex relationships between supply chain components
Optimization is ideal for environments with low uncertainty, where supply chain efficiency is the primary focus. This approach is better suited when looking at a long-term horizon, and where stakes are high (i. e. strategic and high level tactical planning). However, when the uncertainty is very low, optimization can even be extended down to low level tactical planning like inventory optimization. Simulation is ideal for rapidly changing environments where firms focus mostly on their supply chain responsiveness. Hence, it is primarily used for short planning horizons (i. . operational planning). Simulation enables planners to perform what-if analysis in a rapidly changing environment. Modeling approaches are different for simulation and optimization. For instance during optimization of a network planning model, a base model is initially created. This model is then optimized based on the given demand. Different network scenarios are used to change the
base model. The scenario that offers a combination of the lowest network cost and highest service level is taken as the optimum network solution.
The simulation approach for network planning creates a model that represents network behavior over a specific time period. Time is simulated using an event calendar, where individual demand, production runs, order fill operations, and shipments are all scheduled and in turn triggers other future events in the model. Uncertainty of demand is incorporated by using statistical distributions, which eloquently represents a real world scenario. Simulation enables the decision maker to see the impact level of various day-to-day variables on the overall supply chain performance (e. g. dentifying the weeks where the plant might face stock outs). Optimization always has an objective function such as cost minimization or revenue/profit maximization. In case of simulation, the focus is more on observing supply chain behavior over a period of time with the intent to identify the controlling factors of a system and their effect on future performance of a supply chain. Optimization tools focus on giving the best results under certain constraints, such as finding the optimal inventory level to meet a certain target service level. It gives the optimized operating variables as output.
Simulation enables the user to vary a few variables at specific time bucket in the planning period of the modeled scenario. Simulation provides the flexibility of defining variables closer to real life, such as selecting a demand pattern from a wide range of available distribution such as Normal, Poisson, Beta, Max Extreme etc. Supply chain modeling can be further categorized into four segments based on the available alternatives and complexities
of the chain.
Conclusion
With the advancement of technology solutions in the supply chain management pace, it’s possible to utilize the best of simulation and optimization with a single hybrid tool. These tools offer engines which can perform simulation, optimization or combination of both. Simulation engines can be used for high complexity evaluation where numbers of options are few, while optimization is good for low complexity evaluation where available options are numerous such as single period network designing. Combining simulation and optimization can be used for high complexity evaluation and where available options are numerous, such as inventory optimization.
The application of using simulation and optimization can also vary depending on the business requirement. Simulation can be used for baseline validation and optimization can be then used for identifying the optimal network. For the same optimal network simulation can be again used for business level objective validation and what-if analysis. About Accenture Accenture's Supply Chain consulting practice, which has more than 15,000 professionals , works with clients across a broad range of industries to develop and execute operational strategies that enable profitable growth in new and existing markets.
Committed to helping clients achieve high performance through supply chain mastery, we combine global industry expertise and skills in supply chain strategy, sourcing and procurement, supply chain planning, manufacturing and design, fulfillment, and service management to help organizations transform their supply chain capabilities. We collaborate with clients to implement innovative consulting and outsourcing solutions that align operating models to support business strategies, optimize global operations, enable profitable product launches, and enhance the skills and capabilities of the supply chain workforce.
Terms & Definitions Beta distribution: The beta
distribution is a very flexible distribution for modeling probabilities based on Bayesian statistics. It is also used to describe empirical data and predict the random behavior of percentages and fractions. It is a continuous probability distribution. The parameters for the beta distribution are minimum, maximum, alpha, and beta. There are two conditions underlying the beta distribution: 1. The unknown variable is a random value between minimum and maximum 2. You can specify the shape of the distribution as two positive values.
Linear programming (LP): Involves the optimization of a linear objective function, subject to linear equality and inequality constraints. Max Extreme distribution: The maximum extreme value distribution, also known as the Gumbel distribution, is commonly used to describe the largest value of a response over a period of time: flood flows, rainfall, and earthquakes. Other applications include the breaking strengths of materials, construction design, and aircraft loads and tolerances. It is a continuous probability distribution. The parameters for the maximum extreme value distribution are likeliest and scale.
Mixed integer programming (MIP): While solving LP problems if only some of the unknown variables are required to be integers, then the problem is called as mixed integer programming (MIP) Normal distribution: The normal distribution describes many natural phenomena such as IQs, people's heights, the inflation rate, or errors in measurements. It is a continuous probability distribution. The parameters for the normal distribution are mean and standard deviation. There are three conditions underlying the normal distribution:
- Some value of the unknown variable is the most likely (the mean of the distribution).
- The unknown variable could as likely be above or below the mean (symmetrical about the mean).
- The
unknown variable is more likely to be close to the mean than far away.
Of the values of a normal distribution, approximately 68% are within one standard deviation of the mean. Poisson distribution: The Poisson distribution describes the number of times an event occurs in a given interval, such as the number of telephone calls per minute or the number of errors per page in a document.
It is a discrete probability distribution. The parameter for the Poisson distribution is rate. There are three conditions underlying the Poisson distribution:
- The number of possible occurrences in any unit of measurement is not limited to a fixed number.
- The occurrences are independent. The number of occurrences in one unit of measurement does not affect the number of occurrences in other units.
- The average number of occurrences must remain the same from unit to unit.
Stochastic: A stochastic process is one whose behavior is non-deterministic, where a state does not fully determine its next state.
- Accounting essays
- Andrew Carnegie essays
- Automation essays
- Business Cycle essays
- Business Intelligence essays
- Business Model essays
- Business Operations essays
- Business Software essays
- Cooperation essays
- Cooperative essays
- Corporate Social Responsibility essays
- Corporation essays
- Customer Relationship Management essays
- Family Business essays
- Franchising essays
- Harvard Business School essays
- Harvard university essays
- Human Resource Management essays
- Infrastructure essays
- Inventory essays
- Logistics essays
- Management essays
- Manufacturing essays
- Market essays
- Marketing essays
- Multinational Corporation essays
- News Media essays
- Online Shopping essays
- Quality Assurance essays
- Richard Branson essays
- Sales essays
- Selling essays
- Shopping Mall essays
- Small Business essays
- Starting a Business essays
- Stock essays
- Strategy essays
- Structure essays
- Trade Union essays
- Waste essays
- Auction essays
- Balanced Scorecard essays
- Battle essays
- Business Plans essays
- Expense essays
- Income essays
- Intranet essays
- Maintenance essays
- Net Income essays
- Security Guard essays