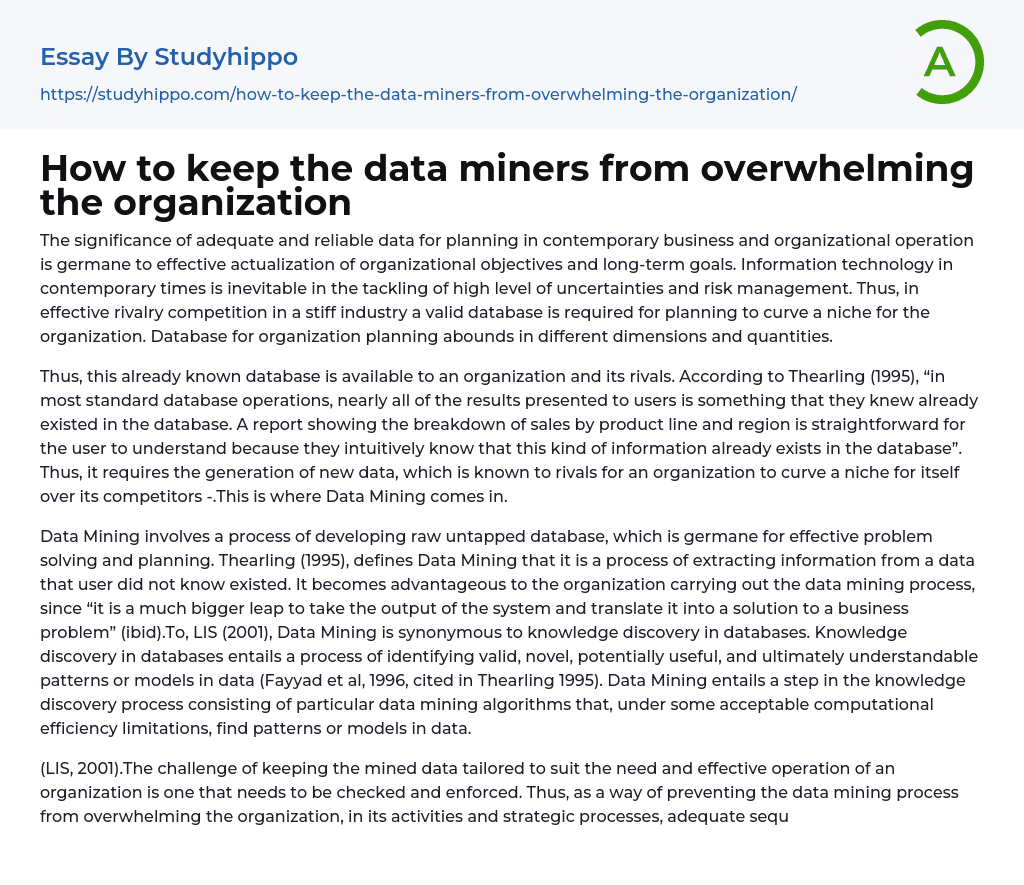
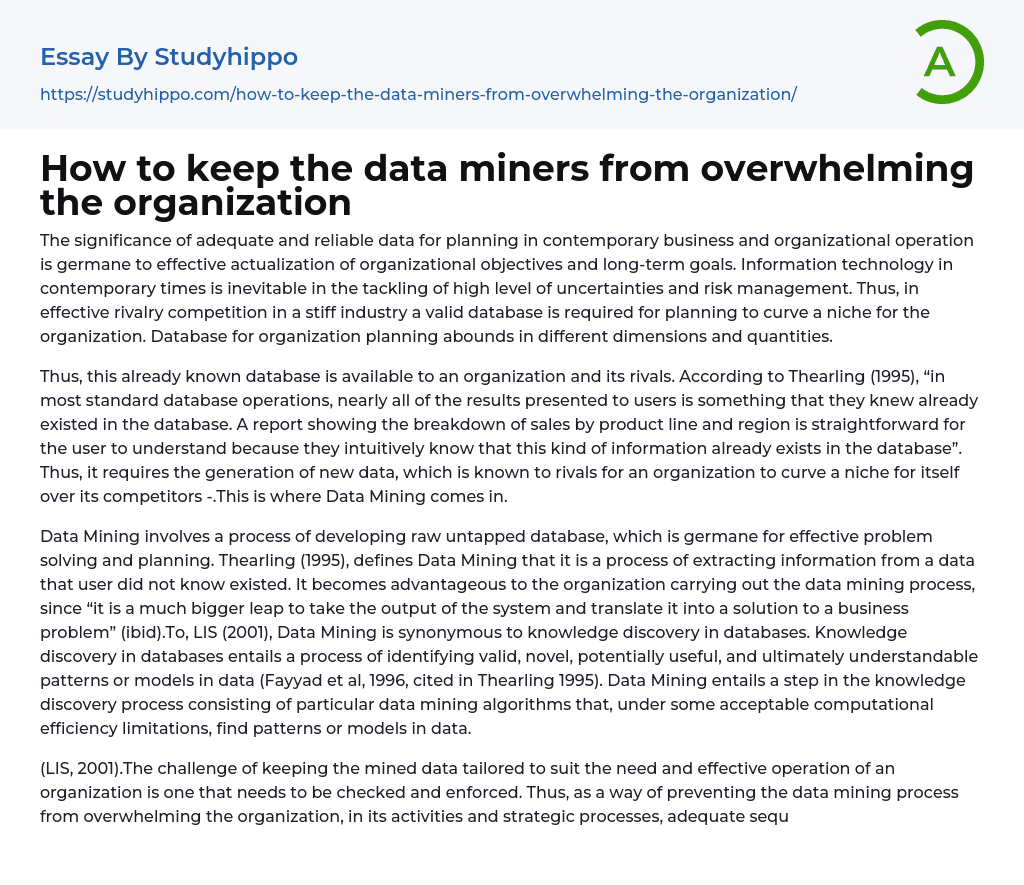
How to keep the data miners from overwhelming the organization Essay Example
The importance of having sufficient and dependable data for strategic planning in modern business and organizational operations is paramount to achieving organizational objectives and long-term goals. The use of information technology cannot be avoided in dealing with high levels of uncertainties and risk management nowadays. Therefore, in order to compete effectively in a highly competitive industry, a reliable database is essential for planning and to carve out a unique position for the organization. Databases suitable for organizational planning come in various sizes and types.
Data Mining plays a crucial role in helping organizations gain a competitive edge over their rivals by generating new data that is not yet known. As Thearling (1995) states, traditional database operations typically present users with information that they already knew existed in the database, such as sales breakdowns by product line and region. To establish a unique position
...in the market, organizations must utilize Data Mining to uncover previously unknown insights.
According to Thearling (1995), Data Mining refers to the process of uncovering information from data that was previously unknown. This process involves developing untapped databases, which is crucial for effective problem solving and planning. Data Mining provides advantages to organizations by assisting in the translation of system output into a solution for business problems.
LIS (2001) equates Data Mining with knowledge discovery in databases, which involves identifying valid, novel, potentially useful, and ultimately understandable patterns or models in data. Thearling (1995) notes that Data Mining is a step in the knowledge discovery process that involves specific algorithms which find patterns or models in data within acceptable computational efficiency limitations.
(LIS, 2001). To prevent the overwhelming effects of the data mining process on
an organization's activities and strategic processes, it is necessary to establish and enforce measures for tailoring the mined data. Adequate sequencing of the data mining process is crucial and will be explored further in this essay.
It is important to exercise caution when engaging in data mining processes for clients. If done in a way that overwhelms an organization's strategic plans and operations, the differing perspectives and targeted aims of the data mining and the organization's strategy can obstruct successful outcomes. Data mining is utilized by organizations for various purposes such as fraud detection, credit management, financial operation management, web mining, etc. These purposes are intended to assist in achieving the organization's objectives and long-term goals. However, if data mining overshadows or contradicts the operations of an organization, it can result in a waste of resources and time that contributes nothing towards actualizing organizational goals. Lavrac et al (2004) assert that for data mining to be successful, it must generate insights that are substantially deeper than what the company already knows about its business.
Before assessing the viability of a data mining approach, designers and analysts need to learn about the client's situation. Additionally, data miners must deeply understand the organization they are working for to avoid overwhelming the organization with the data mining process, which can lead to negative consequences such as ineffective operation. Overwhelming the organization can also result in poor virtualization of data mining output and prevent effective interaction and synchronization between the organization's planning process and users of mined data.
According to LIS (2001), the objectives of an organization's regular problem-solving approach and data-mining approach differ. For example, while the regular approach may focus
on increasing sales, the data-mining approach aims to identify customer attributes related to their purchasing capacity. Similarly, when the regular approach targets mitigate credit card fraud, the data-mining approach emphasizes identifying crucial usage patterns for fraudulent cards and developing precise algorithms for fraud detection. If data mining takes precedence in an organizational context, it could obscure regular objectives and reduce the emphasis on regular problem-solving goals.
Typically, data mining is utilized by data miners to support an organization in reaching its goals and objectives. However, excessive use of the process can lead to opposing actions and hinder the organization's regular operations. To prevent this, data miners must comprehend the organization and its operations. According to Lavrac (2004), "Designers and analysts have to learn a good deal about the client's situation before they can assess the viability of a data mining approach. Successful data mining projects preferably should be done by consultants who already have a deep understanding of the client's business." With massive amounts of data to work with, data miners must fully understand their clients to produce effective mined data that does not overwhelm the organization or its operational activities.
According to Soulie (2006), the abundance of data in modern times is closely tied to the use of data mining applications. In recent years, companies have heavily invested in creating large data warehouses that can reach sizes ranging from 20 to 100 Tbytes and include millions of customers and thousands of variables. These sizes typically triple every two years. To avoid overwhelming organizations, it is crucial for data miners to have a proper understanding of their organization's objectives and long-term goals. This will allow
them to determine which data mining pattern would best enhance operations while ensuring that they achieve their goals and objectives effectively. Conducting a pilot study before implementing data mining applications on organizational operations is another option to prevent organizations from being overwhelmed by these activities.
Data mining is a tool that organizations use to support their strategy and bring about effective operations. It is not a solution to an organization's problems, but it empowers the organization to achieve its goals through vibrant strategies. It is advisable to use a pilot study to ensure that the adopted data mining model is compatible and effective in aiding the organization's operations. Lavrac (2004) recommends starting with one or more small pilot projects with a team consisting of a data mining expert and a domain expert with technical support before embarking on a long-term data mining project.
It is crucial for a small, manageable team to conduct a pilot study to determine effective data mining techniques that empower organizations to tackle their issues without overwhelming them. Choosing the appropriate data mining tool is critical for efficient processing that aligns with organizational structure and specific challenges.
So, in order for data miners to provide effective solutions for their clients, each client's needs must be thoroughly examined and the appropriate database tool must be applied. To select the right data mining model for an organization, LIS (2001) suggests considering "the main task of the project and its relation to main divisions of data Mining tools according to the type of the problem." Therefore, the DM modeling tool should be chosen based on whether the goal is prediction or description, as stated by the same
source (ibid).
In summary, Data Mining involves the use of statistical data and techniques to create a database that helps an organization with effective planning. By utilizing data mining, an organization can efficiently manage its resources and cope with environmental variables in its industry. It is essential that data miners understand the organization and its problems before processing the database, and that the right DM tool is used along with pretesting through pilot study to prevent overwhelming the organization and its operations.
The source cited is an article titled "Lessons Learned from Data Mining Applications and Collaborative Problem Solving" authored by Nada Lavrac in 2004. It was published on April 11 and can be accessed at http://home.comcast.net/~tom.fawcett/public_html/papers/dmll-intro-ver7.6.
On September 27th, 2006, a PDF document titled "LIS (2001), 'Knowledge Discovery and Data Mining'" was accessed via http://dms.irb.hr/tutorial/tut_applic_ref.php. On August 20th, a workshop on "Data Mining for Business Application" was held at the KDD Conference, where Francoise F. Soulie presented "Data Mining in the Real World: What do we Need and what do we have?"
On September 27, 2006, Kurt Thearling published "Understanding Data Mining: It's All in the Interaction," which can be accessed at http://www.labs.accenture.com/kdd2006_workshop/dmba_proceedings.pdf.
On September 12, 2005, DSStar published an article about interaction, which can be found at http://www.thearling.com/text/dsstar/interaction.htm.
- Android essays
- Application Software essays
- Benchmark essays
- Computer Network essays
- Computer Programming essays
- Computer Security essays
- Computer Software essays
- Cryptography essays
- Data collection essays
- Data Mining essays
- Graphic Design essays
- Information Systems essays
- Internet essays
- Network Security essays
- Website essays
- World Wide Web essays
- Consciousness essays
- Intelligence essays
- Mindset essays
- Perception essays
- Problem Solving essays
- Resilience essays